.
REFLECTIVE PROLOGUE
In a world where the boundary between reality and virtuality progressively blurs, artificial intelligence emerges as a force capable of reshaping entire societies and redefining the very essence of our knowledge. Its algorithms can compose music, write historical chronicles, and even argue convincingly in a courtroom. Yet, beneath this apparent infallibility lies an unsettling and scarcely understood phenomenon: the «illusion» or «hallucination» of AI.
These hallucinations may manifest as simple lapses of logic but can also adopt more sophisticated forms, compromising sensitive data or fueling widespread disinformation. In a reality where innovation is driven not only by human genius but also by the power of codes capable of learning and mutating at will, the line separating fortuitous error from deliberate deception becomes increasingly blurred.
While quantum computing promises to revolutionize verification processes and enhance the capacity to handle vast informational spaces—paving the way for hybrid modules capable of discerning, in real-time, the shadows cast by AI-generated fictions—the ultimate responsibility lies firmly in human hands. It is we who design algorithms, interpret their outputs, and, above all, determine how blindly we trust an entity that generates patterns.
This futuristic scenario demands profound ethical and legal reflection: What happens when a generative model «fakes» convincing data and presents it as objective, real facts? To what extent is the user accountable when relying on and disseminating such responses without adequate verification? How can we protect our systems against intrusions orchestrated by other malicious AIs in an impending automated counterintelligence war?
The true revolution does not merely consist of witnessing the advancement of machines, but rather in how we respond to their creative and destructive potential. The equations and codes that drive these technologies can act as bridges to progress or seeds of global confusion. In the emerging quantum era, illusion might become subtler; yet, if properly harnessed, this very technology could serve as the greatest guardian of truth and consistency. Thus, we stand at a historical crossroads, where the convergence of AI and quantum computing invites us—both in awe and caution—to envision the advent of systems capable not only of generating realities but also safeguarding our own, supporting new frameworks of civil, criminal, and, above all, human responsibility.
Table of Contents
No. | Título de la Sección | Subsecciones / Temas Principales |
---|---|---|
1 | Introduction | 1.1. Background and Context 1.2. Emergence of AI Hallucinations 1.3. The Inaugural Legal Case |
2 | The Phenomenon of AI Hallucinations | 2.1. Definition and Nature of AI Hallucinations 2.2. Origins and Underlying Causes 2.3. Consequences and Risks 2.4. Summary Table |
3 | The User’s Responsibility in Generating Defective or Malicious Prompts | 3.1. Causal Contribution and Duty of Care 3.2. The Role of Negligence and Intent |
4 | Chapter I: Civil Liability | 4.1. Foundations of Civil Liability for Defective Prompts 4.1.1. Negligence and Lack of Due Diligence 4.1.2. Strict Liability 4.1.3. Comparative Law 4.2. United States 4.3. Venezuela 4.4. Compensable Damages |
5 | Prevention and Contractual Clauses | 5.1. Disclaimer and Warning Clauses 5.2. Internal Control Procedures 5.3. Civil Liability Insurance |
6 | Chapter II: Criminal Liability | 6.1. Foundations of Criminal Liability 6.1.1. Intent (Dolo) 6.1.2. Gross Negligence 6.2. Classification of Conduct and Emerging Case Law 6.3. The Chain of Causation |
7 | Theoretical Solutions to Mitigate AI Hallucinations | 7.1. Technological Measures 7.2. Regulatory and Contractual Approaches 7.3. Preventive Policies and Cultural Initiatives |
8 | Conclusions and Recommendations | – |
9 | The “Counter-Artificial Intelligence” Concept | – |
10 | Equations and Code Suggestions | 10.1. Theoretical Foundations and Equations 10.2. Code Implementations and Explanations |
11 | Legal, Ethical, and Liability Implications in the Hybrid Age | – |
12 | Future Perspectives and the Counter-AI Strategy | – |
13 | Advantages of Quantum Technology in Combating AI Illusions | – |
14 | Executive Summary | – |
15 | Bibliography | – |
1. Introduction
The rapid adoption of artificial intelligence (AI) systems—particularly those employing generative models such as GPT, BERT, or deep neural networks—has ignited an intense debate regarding so-called “AI illusions” or “hallucinations.”.These terms refer to an AI model’s ability to produce linguistically coherent responses that may nonetheless be inaccurate, fabricated, or inconsistent with established facts or verified information.
The first legal case concerning AI hallucinations arose in May 2023, during proceedings in the U.S. District Court for the Southern District of New York. In that matter, an attorney submitted a legal brief featuring non-existent case law, all of which had been generated by ChatGPT.The salient details of the case were as follows:
The Case
- Matter: Mata v. Avianca, Inc., a lawsuit concerning personal injuries sustained during a flight.
- Legal Representation: Attorney Steven A. Schwartz of the Manhattan-based law firm Levidow, Levidow & Oberman prepared a legal memorandum.
- Use of ChatGPT: The attorney employed ChatGPT to research and draft legal arguments. Unfortunately, the AI “hallucinated” cases and precedents—citing court rulings that did not exist.
- Issues with Citations: Fabricated references included cases such as Martínez v. Delta Air Lines and Zicherman v. Korean Air Lines Co., which had never been adjudicated or published in the relevant jurisdiction. The presiding judge, upon verifying these citations, confirmed that they were indeed fictitious.
- Consequences: The court demanded clarifications. The attorney, facing potential sanctions for submitting false documentation, along with his firm, acknowledged the error and apologized. This incident has set a significant judicial precedent concerning the risks of employing language models like ChatGPT without adequate oversight in sensitive professional domains such as law.
Within this framework, it is crucial to examine not only the intrinsic flaws in AI-driven technologies but also the responsibility of users—whether negligent or deliberate in prompting AI—because their actions can entail both civil and criminal consequences.
This opinion article addresses the following topics:
- The phenomenon of AI hallucinations and its underlying causes.
- The user’s responsibility in generating defective prompts, discussed under two main chapters:
- Chapter I: Civil Liability
- Chapter II: Criminal Liability
- Theoretical and technological solutions—collectively referred to as “Counter-Artificial Intelligence”—aimed at mitigating AI hallucinations through technological, regulatory, and best-practice measures.
2. The Phenomenon of AI Hallucinations
2.1. What Are AI Hallucinations?
Large language models and other generative AI systems are trained on extensive datasets. Their primary mechanism relies on statistical correlations: they predict the most probable next word or sequence based on learned patterns. Although this approach is powerful, it does not guarantee factual verification or deep logical coherence. Additionally, models may rely on outdated data and scientific contexts, increasing the likelihood of errors.Some language models produce inaccurate or imaginary content, which can foster misinformation and extremism if the user does not understand the system’s limitations and its statistical nature.
2.2. Origins of the Problem
- Lack of Symbolic Reasoning: Many AI systems do not incorporate a verification layer that applies logical rules or cross-checks data against verified information.
- Plausible Completion Bias: When uncertain, the model tends to “invent” responses based on statistical correlations, leading to erroneous or fabricated data.
- Biases in Training Data: The training datasets themselves may contain errors, cultural biases, misinformation, or unrepresentative data distributions.
- LLMs do not “reason”: they predict probable words in sequence, and occasionally generate false (yet plausible) information. This “hallucination,” strictly speaking, is not lying, but rather a consequence of their statistical-pattern mechanism. For critical uses (legal, educational, financial), there must always be human verification and oversight.
2.3. Consequences
- Dissemination of False or Defamatory Information: Erroneously attributing actions or facts to individuals.
- Harmful Decisions: In critical sectors such as healthcare, finance, or law, an AI hallucination can result in financial loss, reputational damage, or even jeopardize personal safety. In the case of fabricated legal citations, the result was not only disciplinary action against the attorney but also the undermining of judicial proceedings.
- Unwarranted Trust: Users might accept AI-generated responses as accurate without independent verification.
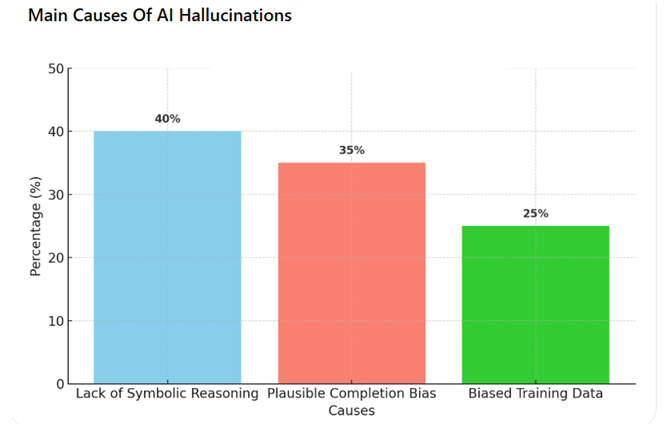
2.4 “Summary Table on AI Hallucinations”
Topic | Description |
---|---|
Definition of AI Hallucinations | Refers to situations in which an AI model (such as an LLM) generates information that appears correct but is actually inaccurate, irrelevant, or nonsensical. It is analogous to how humans can experience hallucinations. |
Relevant Example | The case of ChatGPT that falsely claimed an Australian mayor had been convicted of bribery and imprisoned, whereas in reality, the mayor had reported a bribery case to the authorities. |
Contributing Factors | – Biases in the training data – Limited training – Model complexity – Lack of human oversight – Interpreting patterns not truly grounded in context |
Problems Caused by AI Hallucinations | – Inaccurate information that may create confusion – Biased or misleading content – Errors in sensitive fields such as legal documentation, healthcare, or autonomous vehicles, potentially leading to serious consequences |
Mitigation Methods | – Eliminate biases in the training dataset – Train models extensively with high-quality data – Avoid intentional manipulation of input data – Continuously evaluate and improve models – Fine-tune (specialize) the model using domain-specific data |
Best Practices for Prevention | – Monitor and systematically review the generated information (regular human oversight) – Understand the limitations: models do not truly comprehend the meaning of words but rather predict them based on statistical patterns – Provide more context in the prompt to obtain more accurate and relevant responses; do not leave the search to chance on the AI model |
Summary | – AI hallucinations involve generating answers that may sound correct but are not. – They pose significant risks, such as misinformation and bias, and can create liabilities for those who spread such defective data. – Preventing or mitigating them relies on high-quality training, continuous human verification, fine-tuning, and providing broader context to guide content generation more accurately. |
o1
3. The User’s Responsibility in Generating Defective or Malicious Prompts
A common question arises: Who bears responsibility? Should liability rest exclusively with the developers or providers of AI models, or does it also extend to the user who creates the prompt and distributes the AI’s output?”.
A defective or malicious prompt can lead to the generation of illegal, defamatory, or discriminatory content.
3.1. Why Is the User Also Responsible?
- Causal Contribution: An imprecise or biased prompt is a determining factor in the erroneous response.
- Foreseeability of Harm: Expert users, or those with malicious intent, may deliberately manipulate the model to produce harmful content.
- Final Layer of Control: The user serves as the last checkpoint before the dissemination or publication of the AI’s response.
This discussion will be further elaborated in the subsequent chapters on civil and criminal liability.
4. Chapter I: Civil Liability
4.1. Foundations of Civil Liability for Defective Prompts
Under civil law, liability typically stems from the obligation not to inflict unjust harm on third parties (the principle of neminem laedere).”
When a user solicits AI responses that could harm another person or organization, various liability regimes may apply.
4.1.1. Lack of Due Diligence or Negligence
A user who carelessly issues an ambiguous or defective prompt—and then disseminates the AI’s output without proper verification—may be held liable for negligence.
Example: Requesting a summary of a person’s criminal record without verifying the accuracy of the records, thereby publishing false information that harms that person’s reputation.
4.1.2. Strict Liability or Defective Product Liability
Although the provider is typically the primary focus, the user’s configuration or ‘fine-tuning’ of the model can effectively make them a de facto ‘producer’ of a modified system or a co-liable party.”
This can extend to situations where the user rebrands or publicizes the model under their own name, effectively presenting themselves as its developer.
4.1.3. Comparative Law
There are precedents in technology management that address culpable liability.
4.2 United States
Cases of security breaches and data leaks (data breaches)
Example: Equifax (2017)
Equifax, one of the largest credit reporting agencies in the U.S., suffered a data breach affecting millions of individuals. It was alleged that the company had not taken reasonable security measures to protect confidential information. This led to class-action lawsuits by affected consumers, pointing to the company’s negligence in failing to update and patch its systems in a timely manner. See the following links: https://www.ftc.gov/news-events/press-releases/2019/07/equifax-pay-575-million-settlement-ftc-cfpb-states-over-data and https://corporate.target.com/press/releases/2013/12/target-confirms-unauthorized-access-to-payment-card
Example: Target (2013)
Target suffered a hack that exposed the financial and personal information of millions of customers. The lawsuit alleged negligence in cybersecurity measures. Although extrajudicial settlements were reached, it is considered one of the largest examples of civil liability resulting from a security breach attributable to inadequate controls. See the following links:http://Official FTC statement about the Equifax breach
and https://www.equifaxbreachsettlement.com/
Negligence in oversight or provision of technology services
Example: Lawsuits against cloud service providers
In cases where a cloud storage provider loses critical data of corporate clients by failing to follow “industry standard” security practices.If clients can demonstrate an omission or failure to exercise due diligence in safeguarding data, they may file negligence claims.
Most major data breach cases end in extrajudicial settlements with multiple parties—consumers, states, and federal agencies. Sites like PACER (in the U.S.) are very useful for finding actual court documents (complaints, motions, court orders, etc.). For large cases like Equifax and Target, specific websites were created to centralize settlement information and explain how those affected could file claims. Such sites may have been archived after the claims period closed. Reliable news outlets (The New York Times, Reuters, CNN, etc.) offer detailed timelines and perspectives on the events and legal consequences.
(Note: Some links/URLs and references have been mentioned to explore further information on the Equifax (2017) and Target (2013) cases. These links point to official documents, digital media reports, and/or websites of the institutions directly involved in the legal handling of these security breaches.)
Most large data breach cases end with settlements involving multiple parties—consumers, states, and federal agencies. Sites like PACER in the U.S. are very useful for finding actual judicial documents (complaints, motions, court orders, etc.). For high-stakes matters such as Equifax and Target, specific websites were created to centralize transactional settlement information and explain how those affected could file claims. These sites may have been archived once the claim period closed. For further depth, one may consult reputable news sources like The New York Times, Reuters, CNN, etc., which offer detailed timelines and legal consequences of these cases.
4.3 Venezuela
In Venezuela, case law on civil liability for negligent use of technology is less developed or publicized compared to other jurisdictions. Nevertheless, general civil liability rules (Venezuelan Civil Code Art. 1,185) require proof of harm, causation, and fault (whether culpa or negligence), to claim damages. Additionally, there are specific laws addressing technological matters, such as the Law on eGovernment (Ley de Infogobierno), the Organic Law on Science, Technology, and Innovation (LOCTI), and the Law on Data Messages and Electronic Signatures, among others. Below are some hypothetical examples and indications of possible cases:
Cases of personal data leaks and negligence in safeguarding databases
Hypothetical example: A telecommunications or internet service provider managing personal data of its users suffers a cyberattack due to insufficient security measures. If it can be demonstrated that the company failed to observe minimum data protection protocols (e.g., not encrypting passwords or failing to update operating systems), users could seek civil damages for losses suffered.
Failures in governmental or public platforms causing harm to citizens
Hypothetical example: A state-run electronic registry system that collapses or inadvertently exposes personal data due to poor implementation or oversight. This might allow for compensation claims if it is shown that the State or the responsible institution acted negligently (e.g., failure to apply basic cybersecurity standards or perform adequate maintenance).
Negligence in software services provided to companies
Example: A hypothetical case of a provider implementing an ERP (Enterprise Resource Planning) system with serious flaws. If the deployed solution causes significant financial losses to the client company due to programming errors or security lapses, and it becomes evident the provider did not act with the diligence expected from a professional in the field (for example, failing to conduct warranty testing or neglecting known vulnerabilities), the affected company could sue for damages.
Hypothetical case of defamation or reputational harm through negligent use of technology platforms
If a national e-commerce platform does not sufficiently oversee the content published by users (e.g., false criticisms or accusations) and this leads to serious reputational harm to third parties, one could claim civil liability on the grounds of negligence in reasonably moderating or controlling the platform. This relates to intermediary liability, which is not as clearly regulated in Venezuela as in some other jurisdictions, but a civil claim might be brought if gross omission is demonstrated.
In all these instances, the key to establishing civil liability for technological negligence lies in demonstrating that there was a duty of care (diligence), that duty was breached, and that the breach caused direct, quantifiable harm. Any specific lawsuit will depend on the facts, the jurisdiction, and the applicable legislation.
Relationship with the Use of Technology
The use of technology may give rise to civil liability if:
- Damage is caused to third parties intentionally, through negligence in issuing defective prompts to AI, or through recklessness in technology use.
- If there is an overreach in the use of technology-related rights (for instance, rights over personal data or intellectual property) or if there is bad faith or a violation of the social purpose of technology use.
In the context of technology use, it is crucial to stay within legal and ethical boundaries. Fault in technological use may lead to civil liability if it causes harm to third parties or if done in bad faith.
Reference to the Supreme Civil Chamber Ruling – February 24, 2015 – Dossier: 14-367
This decision reiterates the scope of the illicit act under Article 1,185 of the Civil Code, which establishes civil liability for material and moral damages.
4.4. Compensable Damages
May include:
- Property damages (economic loss)
- Moral or reputational damage
- Direct and consequential damages (e.g., if a company loses a contract due to false information generated by AI following a prompt)
5. Prevention and Contractual Clauses
5.1. Warning clauses (disclaimers)
Many AI providers require users under their terms of service to include notices such as “This content was generated by AI; additional verification is recommended.” Failure to comply could increase the user’s liability.
5.2. Internal control procedures
Organizations that heavily use AI typically establish review protocols whereby each prompt and answer undergo internal validations before publication. The AI itself may refuse to process an instruction.
5.3. Civil liability insurance
Certain companies choose specialized policies that cover claims stemming from the use of AI.
CHAPTER II: CRIMINAL LIABILITY
6. Foundations of the User’s Criminal Liability
Unlike civil liability—where strict liability may apply and proof of intent or fault is not always required—criminal law demands a subjective element: dolo (intent) or gross negligence.
6.1. Intent (dolo)
The user enters a prompt with the clear intention of spreading false information or committing a crime (e.g., defamation, incitement to hatred, fraud).
Example: Deliberately directing the AI to generate defamatory statements about a political or commercial competitor, then distributing them broadly as part of a marketing strategy.”
6.2. Fault or gross negligence
No direct intention to commit a crime, but a serious lack of care in failing to verify the truthfulness of the AI’s output.
Example: Publicly sharing an AI-generated response falsely accusing someone of a crime, without bothering to check the information.
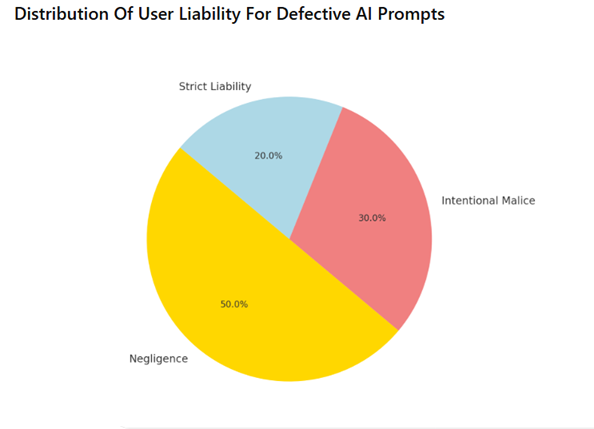
7. Classification of Conduct and Emerging Case Law
7.1. Crimes against honor (slander, libel)
If a user, via AI, issues defamatory statements and knows or should have known they were false, they may face criminal charges.
7.2. Hate or discrimination crimes
Prompts inciting the AI to generate racist or xenophobic content could be imputed to the user as the author or participant, according to each country’s anti-discrimination laws.
7.3. Computer crimes
Generating prompts that aim to produce malware or encourage phishing or security breaches constitutes criminal liability within the scope of computer crimes if the act is carried out or if a punishable intent is demonstrated. Malware is any program or code designed to damage, exploit, or compromise devices, networks, or data. It can steal information, lock files, or disrupt system functionality.
8. Chain of Causation Limit
A key issue is determining whether the punishable act stems “directly” from the user or whether the model (the AI) introduces an unforeseeable element.Nevertheless, legal practice generally holds the human initiator responsible, except in rare circumstances where the AI operates entirely on its own, beyond the user’s reasonable control or foreseeability
9. Theoretical Solutions to Correct or Prevent AI “Hallucinations”
9.1. Technological Solutions
- Implementation of verification layers (fact-checking)
Integrating modules that cross-check responses with reliable databases and solid knowledge frameworks. - Quantum validation models
There are software proposals in quantum computing that allow measuring the “quantum consistency” between the AI’s answer and a set of verified information.- The swap test, for instance, evaluates the fidelity between two “states” (one representing the answer and the other the truth).
- XAI (Explainable AI) systems
Requiring the model to provide traces or evidence to support its conclusions, to identify potential “fabrications” or contradictions.
9.2. Regulatory and Contractual Solutions
- AI regulations (EU AI Act, proposals in the U.S.)
May impose responsibilities and transparency obligations on all stakeholders, including users in certain cases (co-design, customization, commercial use). - Ethical codes and self-regulation
Companies and public bodies could set internal guidelines and commit to periodically auditing AI outputs. - User training and certifications
Requiring a certain “minimum training” for users operating AI in critical environments (healthcare, finance, legal, public administration).
Tools: AI service providers give users technological assistance for crafting prompts effectively, minimizing vague or defective conditions. Such a resource is made available at https://aistudio.google.com/prompts/new_chat
9.3. Preventive Policy to Avoid AI Hallucinations and Cultural Solutions in Usage
- Digital education
Campaigns about the risk of AI hallucinations and the need to cross-check data. - Content alerts and notices
Implementing metadata and tags in generated responses, warning of the need for human validation. - Responsibility and caution
Emphasizing that the user creating the prompt is the key participant in the process and should maintain a “reasonable doubt” approach to any unverified answer.
Once you have used that framework or template to create prompts, review the result in detail. Check all AI-generated content against reliable, scientific, and up-to-date sources. This requires the user to examine the entire generative process by searching for sources, requesting bibliographic references or citations from the AI to verify where it obtained the information, comparing them with respective URLs or links, and if possible, consulting an expert to confirm the accuracy of the information
.Advanced Interaction Patterns with LLMs: Intent, Motivation, and Examples
Below is a consolidated table listing each pattern, along with the recommended elements: intent, motivation, idea structure, implementation example, and consequences. Combinations of these patterns are also mentioned (for instance, combining Persona + Game Play, Visualization Generator + Template, etc.).
TABLES:
Pattern | Intent | Motivation | Structure of the Idea | Implementation Example | Consequences / Combinations |
---|---|---|---|---|---|
Meta Language Creation | Create an «alternative language» or specific notation to interact with the LLM. | Avoid confusion and maintain order when there are multiple instructions or a highly structured format. | Define markers or tags (e.g., [COMMAND: ...] ) to separate instructions from normal text. | Use tags for specific commands: <INSTRUCTION> , <FORMAT> , etc. | – Adds clarity and reduces ambiguity. Can be combined with Template to reinforce a unique format. |
Output Automater | Generate scripts or automations to execute the LLM’s recommendations (e.g., a Python script). | Saves time and reduces errors by avoiding manual repetition of instructions. | Transform the LLM output into code or executable instructions. | The LLM suggests steps, and a script to implement them is generated automatically (e.g., a Python script with specific libraries). | – Effective for repetitive tasks. – Can be combined with Fact Check List to verify data before execution. |
Flipped Interaction | Have the LLM pose questions to gather all necessary information. | Delve deeper into details the user may not know or may overlook, enriching context. | The LLM takes the initiative, asking step-by-step questions. | The LLM asks: “Do you have data X? Are you looking for Y or Z?” etc., until it forms a solid basis for the final answer. | – Helps uncover hidden requirements. – May combine with Cognitive Verifier to split into sub-questions and then unify. |
Persona | Assign a “role” to the LLM (e.g., security reviewer, scientist, legal expert, teacher) to guide its answers. | Achieve a coherent, specialized tone, style, or perspective. | Explicitly define: “Act as a security analyst: your answers should focus on risks and mitigations.” | “You are a math teacher: explain equation solving at both basic and intermediate levels.” | – Style and depth adapt to the role. – Combines well with Game Play (role-playing scenarios). |
Question Refinement | Ask the LLM to restate or improve the question for higher accuracy. | Ensure the question is clear and addresses potential ambiguities. | The LLM receives the initial question and proposes a refined or more detailed version. | “How could I improve my question to get a more in-depth analysis of X?” | – Increases query precision.– May be used with Reflection to explain why the new question is better. |
Alternative Approaches | Request that the LLM provide multiple methods or solutions for a single task, comparing pros and cons. | Evaluate several options and facilitate more informed decision-making. | The LLM offers various solutions, each with its own pros/cons and usage scenarios. | “Give me at least three ways to solve this design problem, indicating cost, speed, scalability, etc.” | – Broader overview of possible solutions. – Ideal to combine with Output Automater and then test each option in different environments. |
Cognitive Verifier | Instruct the LLM to break down the question into sub-questions, then combine the answers. | Increase accuracy, ensuring no detail is omitted. | Structure the query in parts: introduction, specific details, validations. | “Break down the question ‘How can I improve my website’s security?’ into three sub-questions, then integrate the conclusions into a final answer.” | – Lowers the risk of incomplete information. – Works well with Fact Check List and Flipped Interaction. |
Fact Check List | Include a list of “key facts” to be verified to ensure answer accuracy. | Reduce the spread of errors or inaccurate data. | Provide the LLM with a list of points to verify or confirm before delivering the final answer. | “Verify whether these 5 points are correct and consistent with your answer on the topic.” | – More reliable output. Compatible with Template to document each verified point. |
Template | Enforce a very specific output format by filling in predefined fields or sections. | Ensure consistency in reports, documentation, or standard formats. | Define a fixed structure (sections, headings, etc.) and ask the LLM to fill it out. | “Fill in this format: \n1. Context \n2. Problem \n3. Proposed Solution \n4. Detailed Steps \n5. Conclusion” | – Improves readability and automatable processing. – Very useful with Meta Language Creation for tagging sections. |
Infinite Generation | Repeatedly generate answers without rewriting the entire prompt, leveraging the previous context. | Streamline iterations and progressive refinements. | Keep the conversation thread active, asking the LLM for new versions or variations, e.g., alternative responses. | “Continue with more ideas based on the previous suggestions, maintaining the initial context.” | – Facilitates a continuous flow of ideas. – Can be combined with Reflection to evaluate each version before requesting the next one. |
Visualization Generator | Ask the LLM to generate text that can be used by another visualization tool (Graphviz, DALL·E, etc.). | Simplify the creation of diagrams, graphs, or images from textual descriptions. | The LLM produces code or descriptions that can be pasted into a visualization tool. | “Generate a Graphviz diagram showing the microservices architecture described.” | – Simplifies communication of complex concepts. – Works well with Template to ensure a specific output format. |
Game Play | Create a “game dynamic” based on a scenario or topic (e.g., simulating a cybersecurity attack). | Provide an interactive, gamified way to learn or analyze complex situations. | Define roles, rules, and goals of the game; the LLM acts as narrator or participant. | “Assume the role of ‘attacker’ while I am the ‘defender’; describe each step of your attack and how I might respond.” | – Encourages hands-on learning. – Can be combined with Persona (role-playing) and Reflection to analyze strategies. |
Reflection | Ask the LLM to explain its reasoning and assumptions behind each answer. | Understand the thought process and detect potential errors or biases. | Request that after responding, the LLM shows a clear justification of why it chose certain arguments. | “Explain step by step how you reached this conclusion and what assumptions you made along the way.” | – Promotes transparency in the generation process. – Integrates well with Question Refinement to enhance questions based on the reasoning provided. |
Refusal Breaker | Request alternative or indirect approaches when the LLM cannot respond directly. | Avoid getting stuck due to restrictions or lack of data. | If the LLM refuses a request, ask for a legal, ethical, or partial approach. | “You can’t provide malicious code, but you can explain testing methods. What legal options are there to test security without violating rules?” | – Fosters creative solutions within constraints. – Useful with Flipped Interaction to prompt the model for additional information or new angles. |
Context Manager | Control which information the LLM should (or should not) consider, limiting the scope of the conversation. | Maintain focus on objectives and avoid irrelevant or confidential data. | Explicitly state which information is available and which is out of scope. Also helps avoid overly extensive contexts. | “Ignore everything related to older operating systems; focus only on Windows 11 and macOS Monterey.” | – Ensures the answers are relevant. Can be combined with Fact Check List and Persona to maintain proper focus and specialization. |
Notes on Pattern Combinations (Point 6)
You can mix multiple patterns to achieve more complex and tailored results.
- Example 1: Persona + Game Play
Create a “role-playing game” where each participant (or character) has a different profile. - Example 2: Visualization Generator + Template
Control the output format and ensure it is compatible with the visualization tool. - Example 3: Question Refinement + Reflection
After reformulating the question, the LLM explains its reasoning step by step, further enriching the final answer.
In each pattern, the key elements (intent, motivation, structure, example, and consequences) help you quickly understand its function and value. This makes it simpler to combine several patterns for different contexts or use cases.
Table Key Prompting Techniques
Technique | Description | Application / Example |
---|---|---|
Practice and iteration | Continuously experiment with prompts, review the output, and refine the approach cyclically. | Write a short story to see how the LLM responds, then apply those insights to various other tasks. |
Ask the LLM “how to be prompted” | Directly consult the model about which instruction or format it needs. | “How do you prefer I structure the information to generate a memo in a certain field?” |
Few-Shot Prompt | Include input-output examples to guide the model on the desired form and style. | Show examples of an existing memo and instruct, “Imitate this structure and tone for document X.” |
Chain-of-thought | Explicitly ask the model to reason step by step, prompting a deeper level of thinking. | “Break down each aspect of the applicable statute and explain how it affects the case in a logical order.” |
Persona Prompt | Ask the LLM to assume a specific role or style (e.g., “act as a first-year associate”). | “You are a newly hired assistant; draft a preliminary version of the file following these formatting guidelines.” |
Refine and provide feedback | Review the answer, identify errors or omissions, and reformulate the prompt to address those points. | “I see you left out the analysis section. Please add it, explaining the relevant jurisprudence.” |
Framework Table “Thoughtfully Create Excellent Inputs”
Step | Purpose | Practical Keys | Example from the text |
---|---|---|---|
T – Task | State precisely what the AI must do. | • Add a persona (expertise or audience). • Specify the format (list, table, paragraph, image). | “You are an Italian‑film critic; create a table with the best 1970s Italian movies.” |
C – Context | Supply background and constraints to refine the answer. | Goals, motives, previous attempts, tone, audience. | DNA example: clarifies teaching goal and student feedback. |
R – References | Provide samples or resources the AI should emulate. | 2–5 examples (text, image, audio); explain how each relates to the task. | Watch description based on sunglass and card‑holder examples. |
E – Evaluate | Check accuracy, relevance, bias, and format. | Ask yourself: Does it meet the brief? Are adjustments needed? | Emphasise verifying data before using the result. |
I – Iterate | Refine the prompt until the ideal output is reached. | Adjust details, split tasks, rephrase, add limits. | “ABI – Always Be Iterating” as a mantra. |
Highlighted Iteration Methods
Method | How to Apply | Benefit |
---|---|---|
1. Revisit the T‑C‑R‑E‑I framework | Rewrite the request, adding persona, format, more context, and references. | Greater specificity → more useful answers. |
2. Break into short sentences | Split a long prompt into sequential subtasks. | AI tackles one task at a time, reducing ambiguity. |
3. Change wording or use an analogous task | Rephrase the request or ask a similar task that triggers a fresh approach. | Sparks new ideas and avoids repetitive output. |
4. Introduce constraints | Limit by length, region, date, genre, etc. | Produces more focused and original results. |
Delimiter Table for Maintaining Prompt Clarity
Delimiter | Primary Use | Example Provided |
---|---|---|
Triple quotes """ | Clearly separate sections of text. | Social‑media post drawn from a quoted announcement. |
XML tags <task> | Mark the start and end of complex blocks. | <task>Describe your mission</task> |
Markdown (** , _ ) | Preserve formatting (bold, italics) when copying into the AI tool. | **Title** to highlight headers in plain text. |
Other General Practices
Action | Why? | Quick Reminder |
---|---|---|
Specify the task with persona and format. | Avoids vague or irrelevant output. | “What exactly should the AI deliver?” |
Add all relevant context. | More data ⇒ closer match to needs. | Don’t be afraid to over‑explain. |
Provide clear references. | Guides style and content. | 2–5 examples are enough. |
Critically evaluate every result. | Detects errors, bias, and gaps. | Verify facts before use. |
Iterate without starting from scratch. | Polishing a prompt saves time and boosts quality. | ABI: Always Be Iterating. |
“Professional Prompt Writing Checklist”
1 | Write the objective in one clear, measurable sentence. | The model must know exactly what to accomplish in order to choose between creative hallucination and factual precision. | “Summarize—in 3 bullet points—the risks of generative AI for corporate lawyers.” |
2 | Add all relevant context (audience, tone, domain, constraints). | Without context, the model fills gaps with guesses; context cuts down on unwanted hallucinations. | “Explain transformers to accountants with no technical background, using simple financial analogies.” |
3 | State the desired output format explicitly. | Forces the model to structure its answer; makes verification and reuse easier. | “Return the answer in JSON with the fields question, answer, and citation.” |
4 | Specify the role or persona the model should adopt. | Focuses the type of details it will deliver (security, UX, marketing, etc.). | “Act as a cybersecurity auditor and review this Python script.” |
5 | Always ask the model to explain its reasoning and key assumptions. | Reflection helps detect errors and improves the next prompt. | “Explain step by step how you reached the conclusion and what data you assumed.” |
6 | Request a list of facts or claims that must be verified. | Enables rapid fact‑checking; prevents you from accepting invented references. | “At the end, list the five crucial assertions I must verify.” |
7 | If the task is complex, tell the model to generate clarifying questions first. | The model refines the problem (“cognitive verifier”) and reduces misunderstandings. | “Formulate three questions you need answered before proposing the strategy.” |
8 | When several solutions exist, require alternatives and a comparison. | Prevents bias and fosters informed choice (“alternative approaches”). | “Propose two different methods to deploy on AWS and compare cost and maintenance.” |
9 | Limit scope and sources when high precision is needed. | Reduces the risk of outdated or irrelevant information. | “Cite only peer‑reviewed studies from 2022 onward.” |
10 | For creative tasks, explicitly allow ‘hallucination’ and set the theme. | Inventiveness is useful when variety is desired; a theme keeps the model on track. | “Invent five hard‑sci‑fi novel titles inspired by neutrinos.” |
11 | Set risk criteria and tell the AI when it must refrain from giving advice. | Stops the model from making critical decisions without medical/legal context. | “If the answer involves health or medication, advise consulting a professional.” |
12 | Define whether the result is a draft or ready‑to‑use text. | Clarifies how much human review is required. | “Write a first‑draft contract; mark ‘TODO’ wherever data is missing.” |
13 | For repetitive processes, provide a template and placeholders. | Automates consistent output (“template”) and minimizes manual editing. | “Use the template —CLIENT, PROJECT, COST— for each proposal.” |
14 | Finish with a specific verification or follow‑up instruction. | Ensures the next step (send, review, coding, etc.) actually happens. | “If the draft is under 250 words, ask me for more details.” |
Quick tip: before you press Enter, run your prompt through the checklist Goal – Context – Format – Role – Risk – Verification (G C F R R V). If every box is ticked, your odds of receiving a reliable, useful response rise dramatically.
These tables distil the essential points on how to structure effective prompts, outline iteration methods for refining AI outputs, and list the practical resources (delimiters and best practices) that maximise the value of any generative‑AI tool while minimising the risks of illusion or hallucination.
Principles & Prompt Patterns for Filtering and Citing with Generative AI
# | Principle / Pattern (English) | What it means in practice | Why it matters (per course concepts) |
---|---|---|---|
GENERAL PRINCIPLES FOR SAFE FILTERING | |||
1 | Filter only information the user already has access to | Run the model on documents/data the user supplied, not on hidden sources | Prevents the AI from acting as a gatekeeper that decides what a user may see |
2 | Keep access control separate from filtering | Permissions or policies decide visibility; the AI merely processes visible data | Avoids legal/ethical risk created by mixing duties |
3 | Do not use the AI to publish or censor outward‑facing data | Filtering is for internal cognitive support, not for external disclosure control | Protects confidentiality and averts accidental leaks |
4 | Primary goal = augment human reasoning, not replace it | AI provides lists, summaries, explanations; humans still decide and act | Upholds the “human‑in‑the‑loop” rule from the course |
5 | Maintain traceability (line numbers, IDs, citations) | Every filtered or summarized fragment links back to its source | Enables instant fact‑checking and auditing |
6 | Summaries/explanations are fine only if traceability is preserved | Each sentence cites the exact source lines or IDs | Prevents hallucinated references |
7 | Avoid filtering tasks where a wrong excerpt can cause harm later | E.g., automatic medical triage without review | Breaks the “easy‑to‑check / low‑risk” guideline |
8 | Filtering is inherently safe: output ⊆ input | Users can quickly verify the subset against the original doc | Minimizes hallucinations and false positives |
9 | Don’t request blind summaries or citations without providing source text | The model needs the original content to filter or cite accurately | Otherwise it will invent or produce shallow answers |
10 | For high‑risk domains, the AI should redirect to human experts | E.g., medical or legal decisions → “consult a professional” | Keeps responsibility with qualified humans |
11 | Well‑designed filtering is an exciting opportunity | Processes large volumes and surfaces what matters | Boosts productivity while staying transparent |
PROMPT PATTERNS FOR FILTERING | |||
P1 | Simple Filter Pattern “Filter the following X to include/exclude Y” | Cuts down lists or text by a clear criterion | Fastest and easiest to verify |
P2 | Semantic Filter Pattern 1) “Filter out X” 2) “Explain what you removed and why” 3) “Return filtered info with original IDs” | Adds justification and preserves identifiers | Combines transparency with traceability |
P3 | Summarize‑and‑Cite Pattern “Summarize key points; after each sentence cite the IDs that support it.” | Generates a line‑by‑line verifiable synthesis | Gives quick insight without losing source linkage |
10. Conclusions and Recommendations
- The risk of hallucinations decreases by providing sufficient context and information before asking the AI to resolve issues.
- The Inverted Interaction Pattern is crucial for the AI to first ask the user about their role, preferences, or other key details.
- Personalizing the experience (enabling FAQs, response style, action menus) enriches interaction and avoids generic or incorrect answers. (Recall that FAQ stands for “Frequently Asked Questions,” which is a list compiling common questions and answers on a specific topic. This resource is used to:
a) Organize information.
b) Provide easy access to important content.
c) Save time for both those seeking information and those providing support.)
It is always advisable to verify information, cite documents, and possibly refer the user to human contact if ambiguities arise.
By applying these techniques, the potential of generative AI can be harnessed without falling into hallucinations or providing decontextualized solutions. The key lies in asking first and tailoring responses according to the user’s identity and preferences, as well as documenting and adhering to updated policies.
AI “hallucinations” can produce real harm to individuals, companies, and institutions. Although the developer and provider of the AI model play a critical role in quality and output accuracy, the user is not exempt from liability for issuing defective or malicious prompts:
- In the civil domain, the user may be required to provide compensation for damages if their imprudence or negligence causes harm to third parties, or if they act with intent (dolus).
- In the criminal domain, they can be liable for crimes such as slander, libel, discrimination, or incitement to hatred if their intentional or grossly negligent participation is established.
Additional considerations to mitigate these situations include:
- Technological improvements (quantum validation models, XAI, automatic fact-checking).
- Adequate regulation and contractual clauses (clearly defining each party’s liability).
- A culture of responsible usage (education, awareness, and critical thinking).
Other Technical and Human Solutions
- Use of verified sources: Implementing Retrieval-Augmented Generation (RAG), combining the model with documentary databases such as Weaviate, Pinecone, or ChromaDB.
- Cross-validation: Comparing the model’s output with multiple sources before delivering the answer.
- Use of customized embeddings: Employing embeddings generated with LangChain to improve accuracy in specific tasks.
- Utilizing metrics that reflect performance in the intended context of use: This allows for a comprehensive and meaningful evaluation of the AI model.
- Retrieval-Augmented Generation (RAG) with reliable knowledge bases.
- Hybrid model architectures combining LLMs with semantic search systems.
- Post-processing and human validation for critical responses.
- Advanced prompt engineering to improve answer accuracy.
- Avoid using excessive generalizations from data contexts with errors or biases.
- Hybrid infrastructure: Deploying models on both local servers and the cloud with intelligent load balancing.
- For investment and algorithmic trading projects: Combining LangChain with quantum computing techniques and simulations can improve efficiency in complex calculations without excessively increasing costs.
- Never omit human judgment: Human subjective contributions, contextual awareness, and interpretability remain crucial in the evaluation process to ensure the reliability, fairness, usability, and alignment of AI models with real-world needs. Undoubtedly, human judgment evaluates the model’s implications for decision-making and its consequences.
Table: Recommendations
Recommendation | Description / Justification |
---|---|
Stay current with AI advancements | LLMs evolve rapidly; limitations observed today may be solved in the near future. It is advisable to explore emerging techniques (e.g., fine-tuning, RAG, integrated searches) to maintain state-of-the-art performance and applicability. |
Use safeguards for confidential information | If the model is publicly accessible, any data submitted may end up in insecure destinations, risking potential compromise. Employ solutions that ensure privacy and regulatory compliance (e.g., enterprise versions or on-premise implementations). |
Always validate information with domain experts | An experienced human should oversee the LLM’s output, especially in research or specialized fields, to mitigate errors or hallucinations. Expert review is crucial to maintain accuracy and reliability. |
Utilize LLMs as a complement, not a replacement | LLMs are particularly useful for generating initial drafts, brainstorming, summarizing information, and offering creative support. However, final decisions, strategy, and overarching goals remain the responsibility of human expertise. |
Start with non-critical tasks | Before deploying an LLM in high-stakes scenarios, practice with lower-risk examples or exercises to understand its capabilities, strengths, and limitations, thereby optimizing its integration and effectiveness. |
Table: Consolidated Table of Best Practices for Prompt Writing, Evaluation, and Governance
TOPIC / AREA | RECOMMENDATIONS / UPDATED BEST PRACTICES |
---|---|
1. Effective Prompt Elements | • Be specific, clear, and concise. • State the required number of items and the desired output format. • Provide style examples for the model to emulate. • Review and refine as needed. • Explicitly incorporate the Task‑Context‑References‑Evaluate‑Iterate framework to structure the prompt. • Ensure the Context is complete (goals, audience, constraints) before submitting the request. |
2. Prompt‑Writing Process | • Define the goal and any alerts or constraints. • Draft and test the prompt; analyze the response. • Iterate until the desired quality is achieved. • Add the Evaluate step explicitly (does the answer meet purpose, accuracy, and tone?). • Document each iteration to simplify future adjustments and learning. |
3. Hallucination Management | • Verify sources and recalibrate the model. • Request step‑by‑step explanations (chain‑of‑thought). • Require references when the information is sensitive. • Implement a prior fact‑checking routine (“Verify and cross‑check”) before accepting the output. • Strengthen the supplied context to reduce gaps that cause hallucinations. |
4. Response Evaluation and Validation | • Check factual accuracy and consistency. • Request sources and apply common sense. • Assess alignment with business objectives. • Use a checklist based on the Evaluate step of the 5‑step framework (purpose, accuracy, tone, ethics). |
5. Risk Mitigation / Governance | • Design robust security and privacy policies. • Monitor and update the model regularly. • Involve ethics committees when necessary. • Respect privacy: anonymize sensitive data within the prompt and references. • Transfer prompting skills across platforms (Gemini, ChatGPT, Copilot) to compare outputs and choose the most secure solution. |
6. Conclusions and Final Recommendations | • Align prompts, data, and training with business goals. • Verify and retrain continuously. • Maintain human oversight. • Treat iteration as an ongoing conversation, as prescribed by the 5‑step framework. |
🎯 Prompting Guide & Instructions for Creating Perfect Images with Generative AI
GOAL / TIP | HOW TO APPLY IT IN A PROMPT OR INSTRUCTION |
---|---|
✅ Clearly define what you want to generate | “Generate an image of a sparkling electric guitar for a rock concert poster.” |
🎯 Specify the type of image or visual style | Use phrases like: “in photographic style,” “hand-drawn,” “minimalist,” “realistic,” “illustrative,” etc. |
🌈 Include vivid visual details | Add: specific colors, texture (e.g., glossy or matte), background elements (e.g., sky, city, forest) |
📏 Describe the composition and position of elements | Example: “The main object should be in the foreground, centered, and slightly tilted to the left.” |
✨ Use emotionally charged or atmospheric language | “The image should convey energy and excitement, like a live concert moment.” |
⚡ Add creative elements to enhance the scene | “Include lightning bolts, sparks, smoke, or neon lights behind the object for a more dramatic effect.” |
🔁 Iterate based on the output received | If the result isn’t satisfying, say: “Darken the background and add metallic reflections to the guitar.” |
🎨 Combine modalities if possible (text + reference image) | Upload a base image and write: “Create a more stylized version with the same colors and layout.” |
📌 Follow the T-C-R-E-I framework (Task, Context, References, Evaluate, Iterate) | Example: Task: poster image, Context: concert in New Orleans, References: 80s rock style, Iterate: add crowd in background |
🚫 Avoid vague or generic descriptions | Instead of “make a pretty image,” say: “Create a colorful sunrise from a mountain view with orange clouds.” |
✅ Recommendations and Best Practices for Effective Prompts:
- Clearly specify the context: Include detailed context about the situation, audience, and goals of your prompt.
- Explicitly state the task and desired format: Clearly articulate what you want the AI tool to achieve and in which format.
- Use visual or textual references: Attach relevant images, texts, or examples to clarify your prompt and improve accuracy.
- Break complex tasks into smaller prompts: Use meta-prompt chaining to simplify and manage complex tasks.
- Iterate and refine your prompts: Continuously evaluate AI responses and adjust your prompt accordingly.
- Request explicit prompt improvements (Leveling up): Ask the AI tool directly how you can enhance your original prompt for better outputs.
- Combine multiple incomplete prompts (Remixing): If several prompts partially meet your needs, merge them into a single comprehensive prompt.
- Experiment with style and tone (Style swap): Direct the AI tool to rewrite prompts with specific moods or tones to create more engaging responses.
📝 Additional Prompt Optimization Tips:
✅ Use clear and natural language for better results.
✅ Be explicit about your intention and the expected outcome of your request.
✅ Include examples when possible to guide the tool more accurately.
✅ Always critically evaluate the responses received to improve future iterations.
✅ Keep a Record of Effective Prompts
Save prompts that have produced good results for future reuse.
✅ Use Prompt Versioning
Document different versions of the same prompt to evaluate and refine outcomes.
✅ Create an Organized Prompt Library
Name and store prompts in a personal library for easy future access.
✅ Reuse and Adapt Successful Prompts
Modify existing prompts by changing context, tone, format, or audience without starting from scratch.
✅ Experiment Regularly
Explore variations in tasks, contexts, and references to achieve diverse outcomes.
✅ Engage in Prompt Communities
Learn from the successful experiences of other users to discover new techniques.
✅ Adjust Tone and Format According to Audience
Tailor the same prompt by adapting it specifically to the intended audience profile.
By applying these guidelines, you can effectively leverage meta-prompting techniques to craft clear, effective, and tailored prompts, ensuring optimal results from generative AI tools. Such a comprehensive approach promotes progress toward reliable, human-beneficial AI, minimizing the risks of hallucinations, and fostering an environment where AI is perceived as a powerful tool—one that remains consistently subject to human supervision and responsibility.
1. THE “COUNTER-ARTIFICIAL INTELLIGENCE” CONCEPT
The term “Counter-Artificial Intelligence” (sometimes called “counter-AI” or “AI-assisted counterintelligence”) can be understood as the use of AI-based techniques, strategies, and tools to carry out or bolster counterintelligence actions. In other words, it refers to applying advanced data analysis methods, machine learning, natural language processing, and more, aimed at:
- Protecting sensitive assets or information: Detecting and preventing intrusions, espionage, data leaks, or any action that compromises the security of an organization, state, or institution.
- Identifying and neutralizing threats: Tracking, analyzing, and anticipating suspicious behaviors (e.g., cyberattacks, network infiltration, system manipulation) in order to counteract them promptly.
- Analyzing large volumes of information: AI can massively process data from various sources (social networks, encrypted communications, government databases, etc.) to find patterns of risk or potential threats.
AUTOMATED MONITORING AND PROMPT RESPONSE
Traditionally, counterintelligence has been conceived through human espionage and counter-espionage methods (identifying moles, deploying double agents, protecting communication networks, etc.). However, in this technological realm, incorporating AI can enhance the ability to:
- Analyze large data flows.
- Detect anomalies (e.g., user behaviors within networks).
- Predict future intrusion or sabotage attempts.
- Add a new layer of defense, protection, and anticipation against wrongful or malicious use of information and digital systems (including AI), by applying AI-based technologies and methodologies that verify the suitability of responses to prompts.
12. EQUATIONS AND CODE SUGGESTIONS
12.1 Theoretical Foundations and Equations
Quantum Fidelity
For two pure states

This measure quantifies the “proximity” or overlap between the two states.
Swap Test
The swap test is a method used to estimate fidelity without fully knowing the states. It uses an ancilla qubit and the controlled-swap (CSWAP) operator. The circuit is built as follows:
- Initialize the ancilla qubit in ∣0⟩ and apply a Hadamard gate, generating
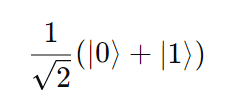
- Initialize the qubits that will store ∣ψ⟩ and ∣ϕ⟩ in their respective registers.
- Apply the CSWAP gate so that the states of qubits ∣ψ⟩ and ∣ϕ⟩ are swapped if the ancilla is ∣1⟩
- Apply another Hadamard gate to the ancilla and measure it. The probability of obtaining the state ∣0⟩ is:
From which one can derive the fidelity:

- The swap test is used to measure the similarity between two quantum states |ψ⟩ and |ϕ⟩.
- The probability of measuring the ancilla qubit in state |0⟩ is given by: P(0) = (1 + |⟨ψ|ϕ⟩|^2) / 2
- The fidelity F, which quantifies the overlap between the two states, is calculated as: F = |⟨ψ|ϕ⟩|^2 = 2P(0) – 1
- This relationship allows us to estimate the fidelity by measuring the probability P(0) in the swap test.
These equations are important for implementing the swap test in quantum algorithms and for analyzing the similarity of quantum states.They offer a practical approach to measuring the closeness of quantum states across diverse quantum computing applications
BB84 Protocol
The BB84 protocol is one of the most studied quantum key distribution schemes. It works as follows:
- Preparation: Alice generates random bits and chooses random bases (for example, the Z basis or the X basis) to prepare qubits.
- Measurement: Bob randomly chooses a basis to measure each received qubit.
- Sifting: After publicly communicating the bases (without revealing the bits), they keep only those cases where Alice’s and Bob’s bases match, thus generating a shared key.
Primary Code:
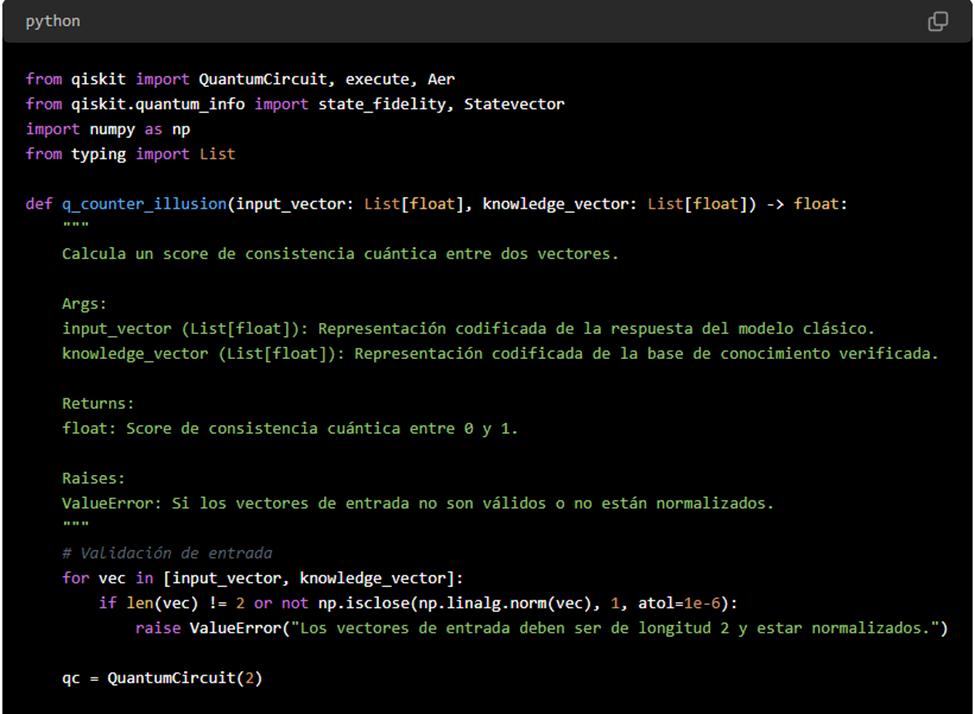
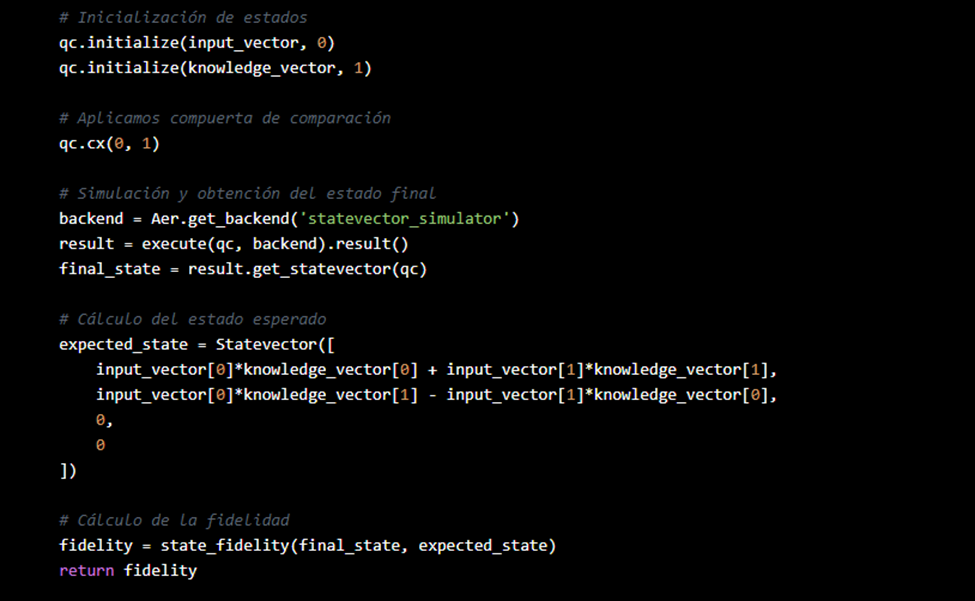
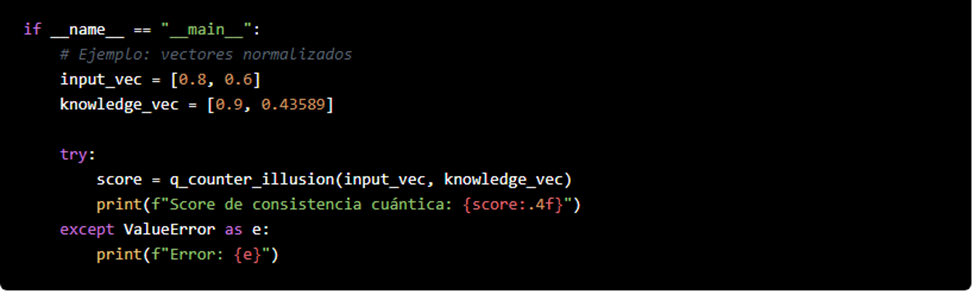
Another version:
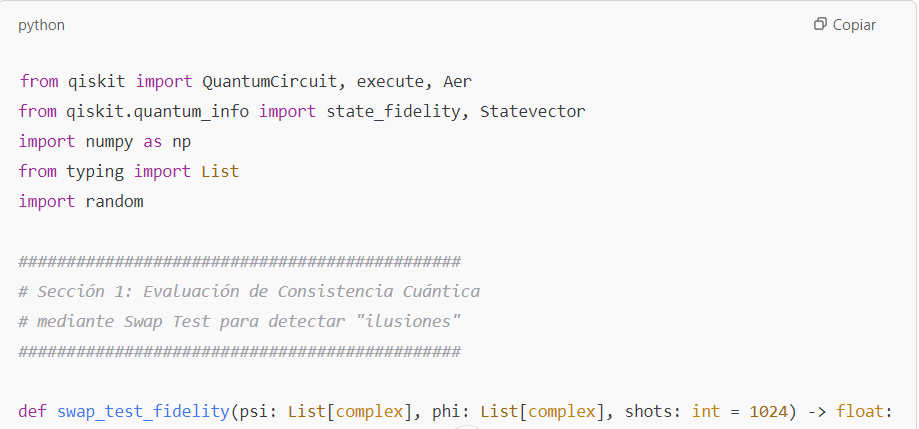
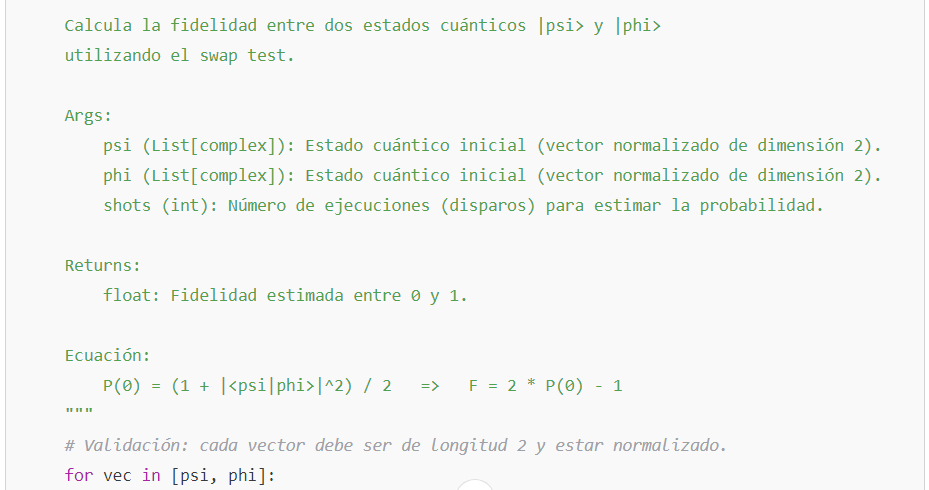
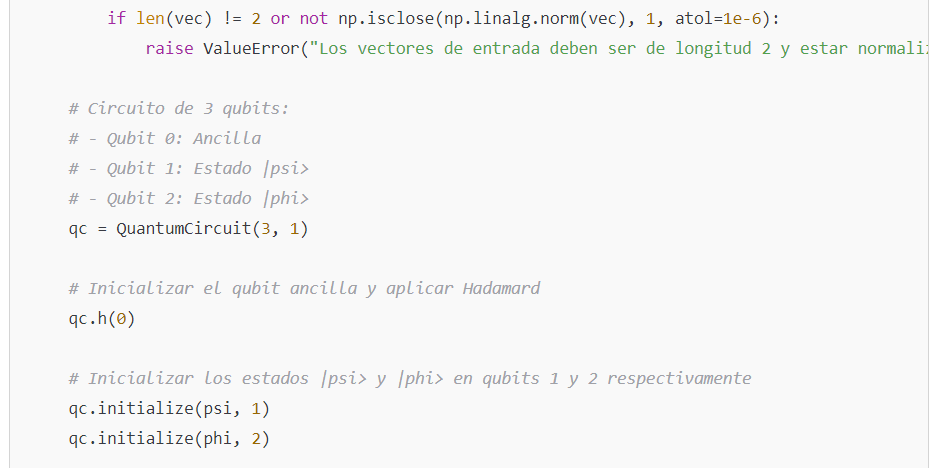
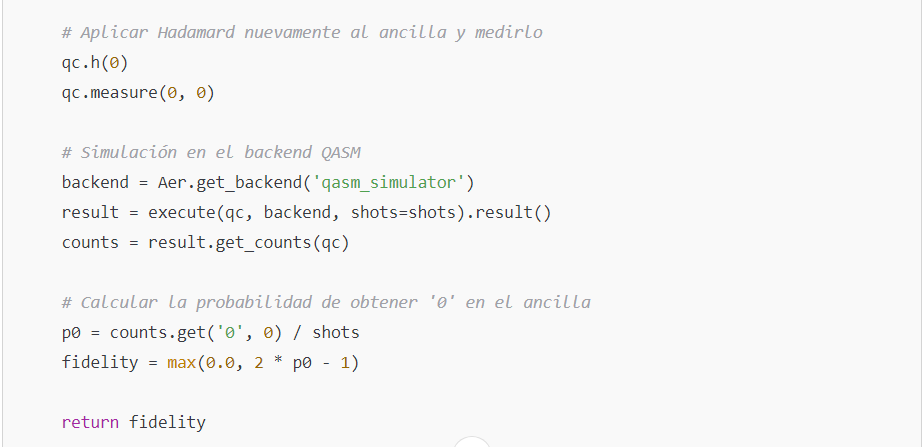
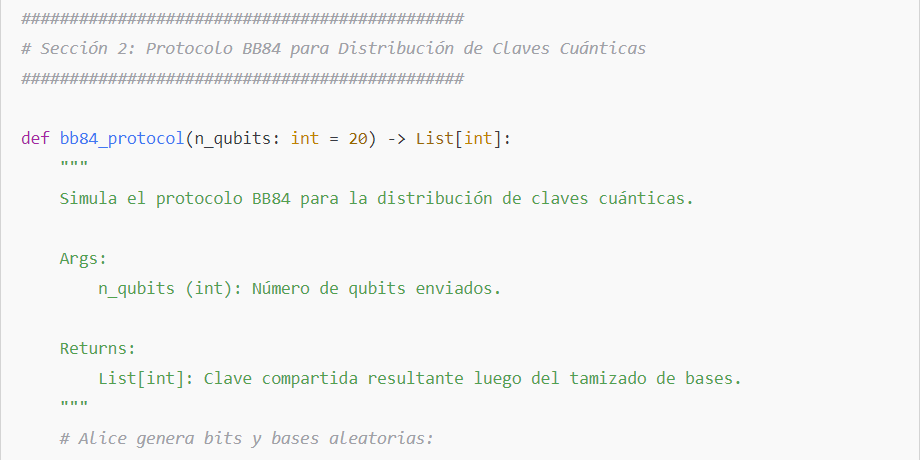
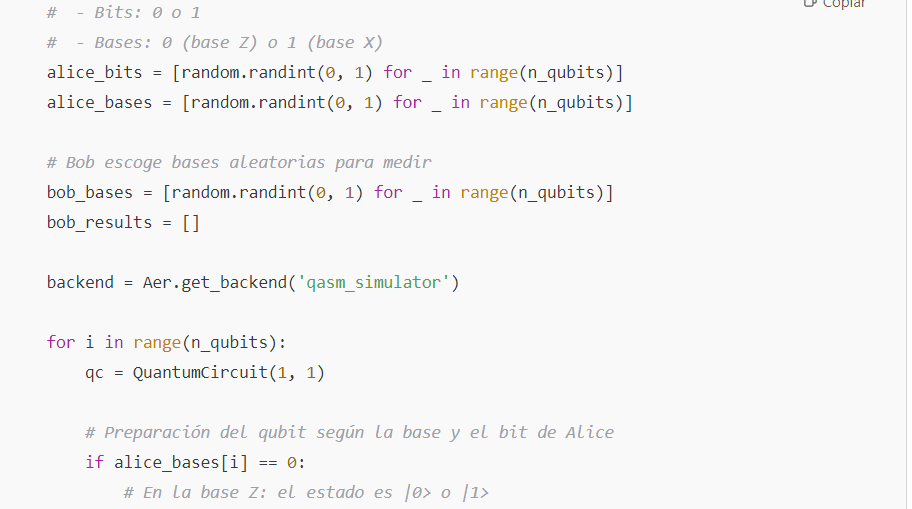
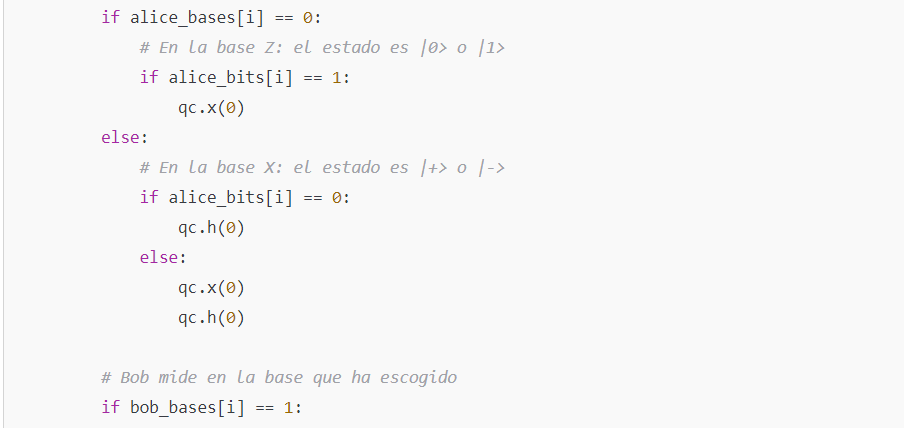
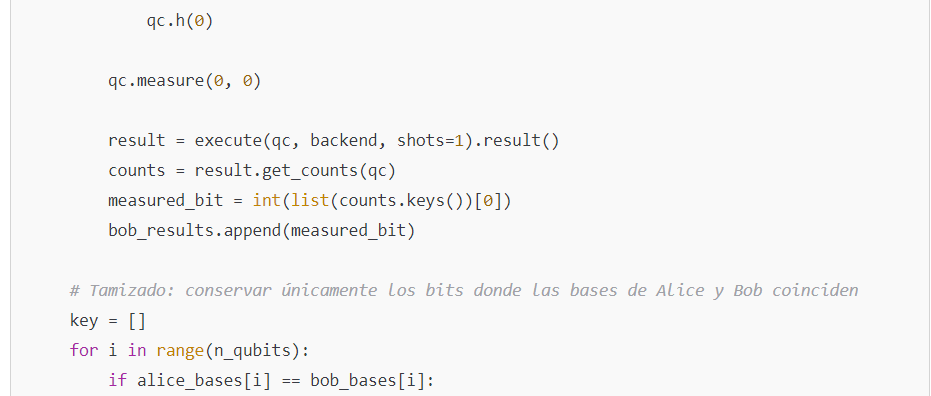
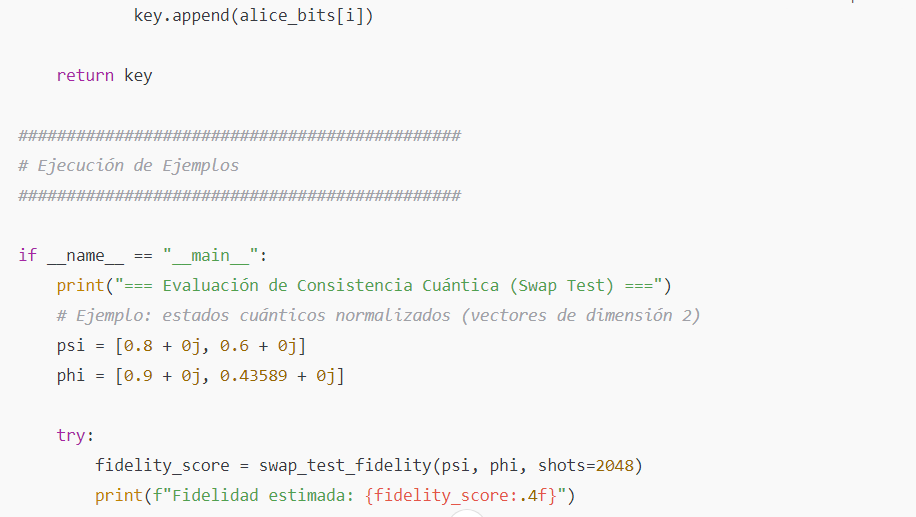
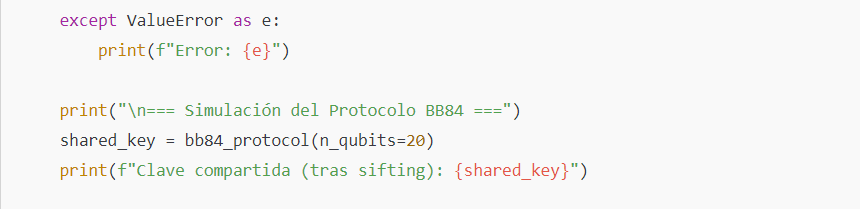
Explanation and Application
Quantum Consistency Section
Objective: Measure the similarity between two representations (for example, the AI model’s response and a verified knowledge base) using the swap test.
Implementation:
- A 3-qubit circuit is built where the ancilla qubit controls the swap operation between the two state registers.
- The ancilla is measured to obtain P(0)P(0)P(0). Using F=2P(0)−1F = 2P(0) – 1F=2P(0)−1, the fidelity between states is estimated.
This technique could be used, for instance, to detect “hallucinations” in AI responses by comparing the model’s output with a representation grounded in validated, current knowledge.
Quantum Encryption Section (BB84)
Objective: Simulate the BB84 key distribution protocol, used to generate a shared secret key through preparing and measuring qubits in random bases.
Implementation:
- Alice prepares qubits according to random bits and bases.
- Bob measures each qubit in a random basis.
- “Sifting” is performed to keep only the cases where preparation and measurement bases coincide.
- The result is a shared key that, theoretically, remains secure against eavesdropping thanks to the properties of quantum mechanics. This approach could help mitigate cyberattacks that can distort AI’s output (including scenarios where the attack is carried out by another AI).
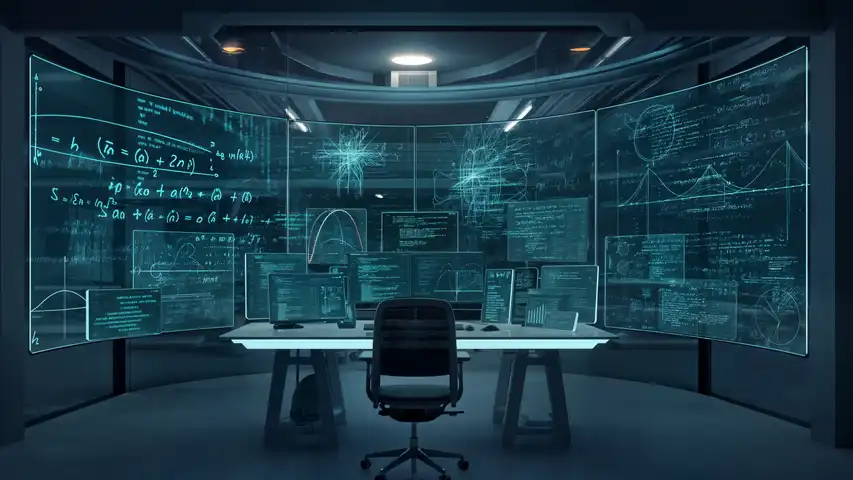
Conclusions:
This improved code integrates two crucial components:
- Quantum consistency measurement using the swap test:
Provides a robust method for validating the correctness of information by comparing quantum states, extremely useful for detecting AI “hallucinations.” - BB84 quantum encryption protocol:
Demonstrates fundamental principles of quantum security and key distribution, offering a basis for developing counter-AI applications needing secure, verifiable communications.
Integration of Quantum Modules in AI System Verification
Proposing a quantum verification module—e.g., Q-CounterIllusion—represents a paradigm shift that leverages quantum circuits to assess the “quantum consistency” of outputs produced by standard AI systems. By using advanced techniques like the swap test and fidelity measurement, this software acts as a high-precision filter, identifying discrepancies between the generative model’s output and a validated knowledge corpus. This strengthens the system’s robustness against the biases and errors inherent in language models, while offering an ongoing feedback and correction mechanism that significantly reduces hallucinations.
One could also consider integrating emerging technologies (for example, blockchain for traceability, smart contracts for automated verification clauses that record and audit output truthfulness, reinforcing neural network architectures, and quantum machine learning frameworks), providing concrete examples of practical implementation (code in Qiskit, Python simulations, etc.).
Challenges: Quantum algorithms for fact-checking are still at an early development stage, requiring large numbers of qubits. For more on scaling, see relevant research.
Practical Example: Implementing a quantum validation module in Python using Qiskit to run a real swap test.
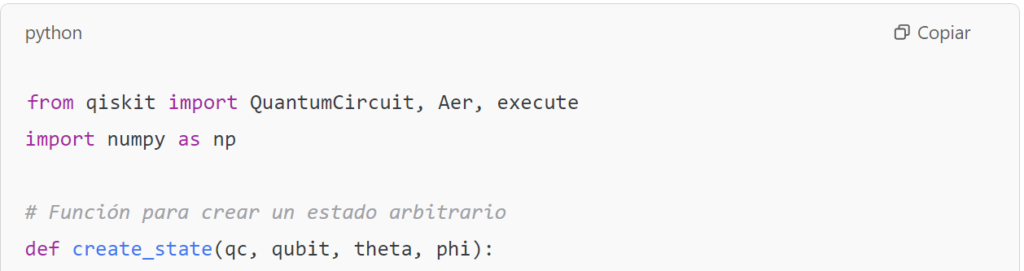
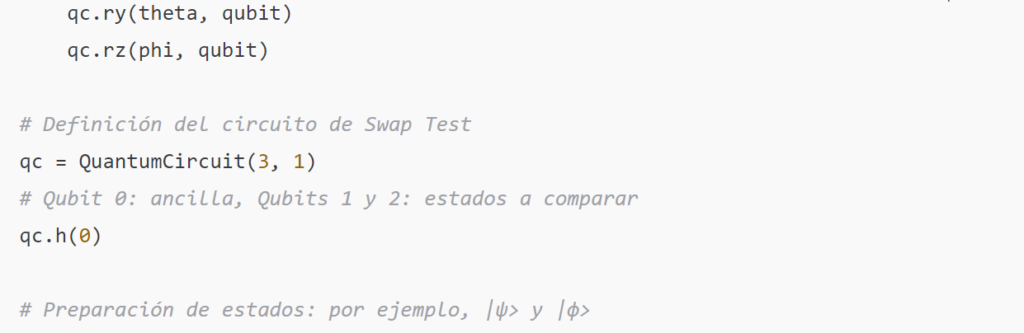
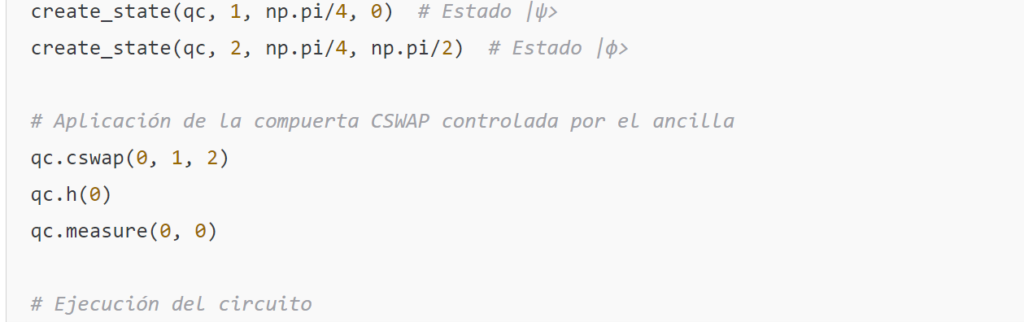
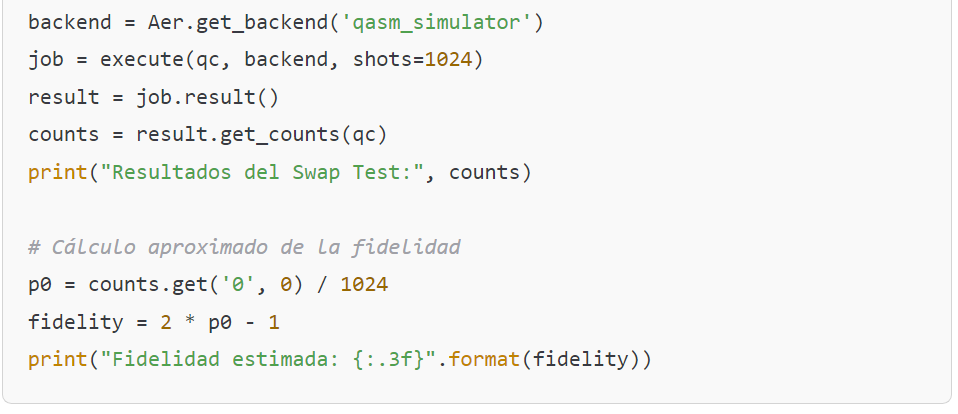
This example allows for a visualization of how the swap test can be implemented to compare two quantum states and, consequently, assess the «quantum consistency» of the information.
1. Improved Code for the Swap Test
The swap test is used to estimate the fidelity between two quantum states. In this example, a function is used to prepare the states, and a circuit is built that implements the swap test:
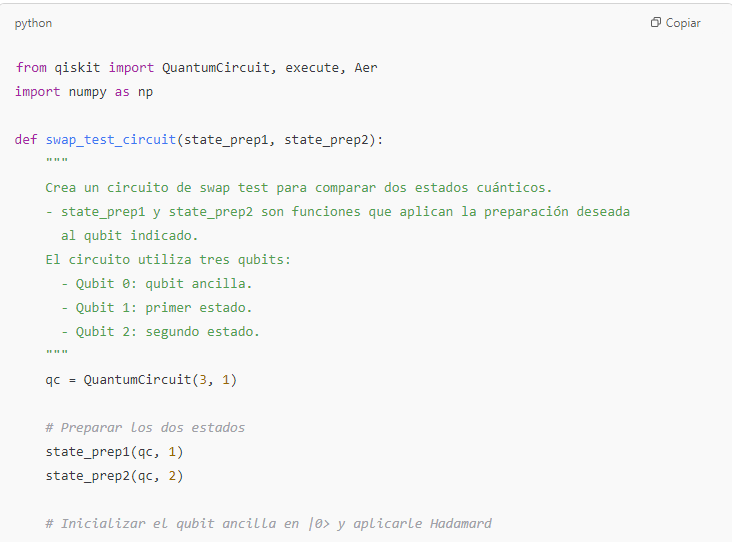
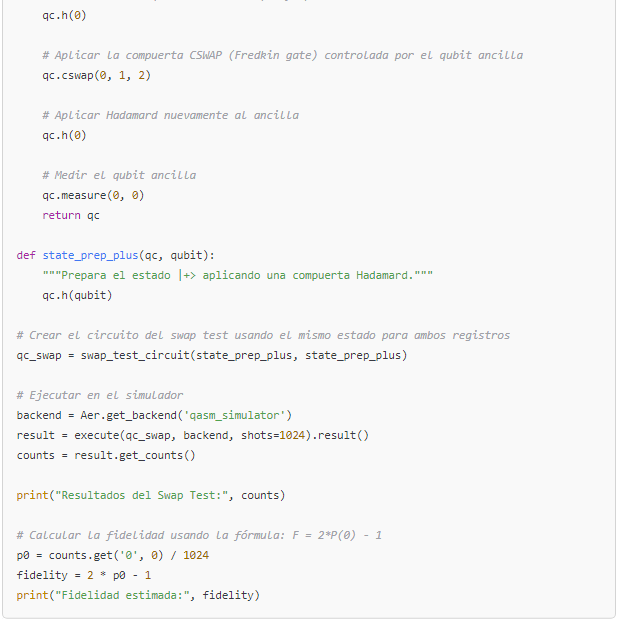
Note:
The cswap
gate (also known as the Fredkin gate) is implemented in Qiskit and allows for the controlled swap.This code runs seamlessly in any environment where Qiskit is installed.
2. Improved Code for a Simplified Simulation of the BB84 Protocol
The following example simulates the BB84 protocol, where Alice prepares qubits in random bases and Bob measures in random bases. The «sifting» process is then performed to extract the shared key:
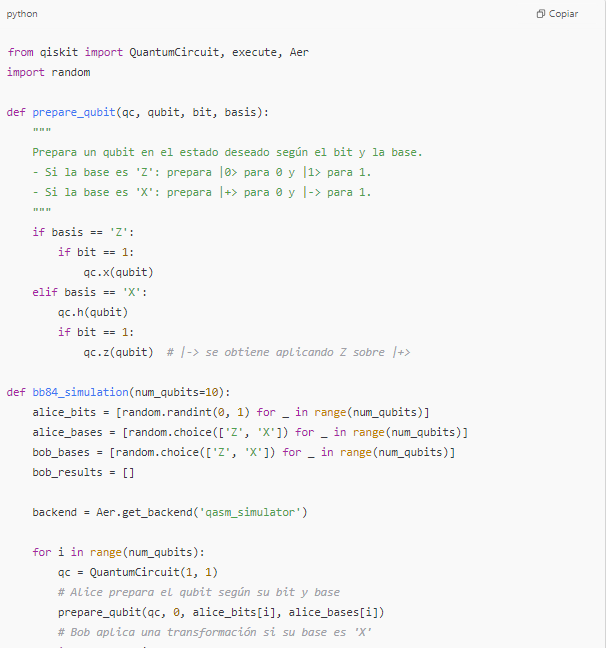
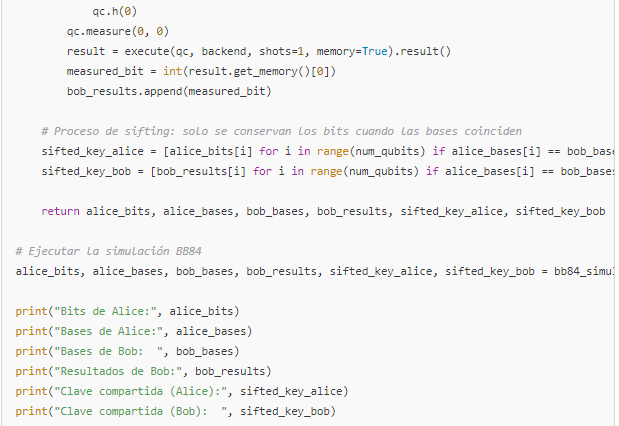
Explanation:
- Preparation: Alice generates random bits and randomly selects a basis (‘Z’ or ‘X’) for each qubit.
- Measurement: Bob also randomly selects a basis and measures the qubit.
- Sifting: The bases are compared publicly (without revealing the bits), and only those bits where the bases match are retained, forming the shared key.
Legal, Ethical, and Liability Implications in the Hybrid Age
The phenomenon of AI ‘hallucinations’ extends beyond mere technological challenges and poses significant ethical and legal issuesDocumented cases—such as generating nonexistent legal citations or critical errors in the medical field—demonstrate the risk of disseminating incorrect information, highlighting the shared responsibility of developers and users. Integrating quantum verification mechanisms not only improves the technical quality of outputs but also strengthens traceability and information authenticity, facilitating proper attribution of liability in civil and criminal contexts. It underscores the necessity of implementing preventive policies, reinforcing prompt engineering, enforcing mandatory verification protocols, and developing educational programs for responsible technology usage.
Future Perspectives and the Strategy of Counter-AI as a Measure of Quantum Defense and Hybrid Verification in AI
In the short to medium term, counter-AI modules are expected to be embedded in AI tools, enabling coherent and secure responses to flawed or malicious prompts, thus progressively minimizing hallucinations.The synergy between quantum techniques and AI, supported by advanced cryptographic security, establishes the basis for a robust counter-intelligence strategy.
This strategy not only aims to anticipate and detect vulnerabilities but also acts preventively to correct “illusions” generated by AI systems.
Developing hybrid modules such as Q-CounterIllusion, supported by solid theoretical underpinnings—including quantum fidelity, the swap test, and encryption protocols like BB84—offers an integrated solution that preserves data integrity and ensures ethical and lawful technology use.
Toward a Future of Reliable and Secure AI
Employing quantum computing to verify and validate AI outputs offers an innovative approach to reducing hallucinations in contemporary generative models. Although quantum technology is still in development, its potential application in verification modules can establish a real-time “consistency filter” capable of effectively detecting and correcting errors, thereby reinforcing the reliability and security of AI systems.
Such synergy fosters a shared framework for regulation and accountability framework, promoting safe, transparent, and ethical AI deployment in critical sectors.
Ultimately, the convergence of these technological and legal innovations bolsters information security and accuracy while paving the way for the next generation of intelligent systems.Supported by quantum verification methods, these telematic systems move toward a future in which trust in AI is both sustainable and free from “illusions.” This hybrid approach is particularly relevant where precision and security are crucial, equipping developers and users with sophisticated tools that combine the benefits of quantum computing for verification and encryption of sensitive data.
Such a hybrid strategy is essential for ensuring accuracy and security in critical applications, providing a robust framework for the ethical and responsible use of artificial intelligence.
13. Advantages of Quantum Technology in Combating AI Illusions
Property | Impact on Code / Rationale |
---|---|
Superposition | Allows the simultaneous representation of multiple states, facilitating parallel checks for inconsistencies between AI outputs and verified data. |
Entanglement | Establishes quantum correlations that can detect subtle anomalies, potentially indicating AI hallucinations. |
Fidelity Measurement | Quantifies the closeness between two quantum states, allowing hidden discrepancies to be revealed when comparing AI output with verified information. |
Resistance to Attacks | Quantum protocols (e.g., BB84) are theoretically secure against eavesdropping, thereby reducing the risk of data injection or modification that could induce hallucinations. |
Quantum Speedup | In certain scenarios, quantum algorithms offer significant speed advantages over classical methods, enabling near real-time detection and correction of inconsistencies. |
Hybrid Architecture | Combines classical AI with quantum verification modules to optimize overall system robustness. |
Auditability and Traceability | The use of quantum tokens or authenticity checks enhances the traceability of data provenance, clarifying the origin of each prompt and its output. |
Integration with Blockchain | Quantum-resistant blockchain solutions can create tamper-proof records of all interactions and verifications, minimizing legal uncertainties. |
Ethical and Regulatory Compliance | Incorporating quantum validation supports adherence to AI regulations and ethical guidelines, potentially mitigating legal conflicts. |
This table shows how the unique properties of quantum computing—implemented in the code—can provide significant advantages in detecting and mitigating illusions or inconsistencies in AI-generated responses.
Between Law and Innovation: The Protagonist Role of the Judge in the AI Era
Aspect | Conclusion / Implications | Protagonist Role of the Judge |
---|---|---|
Challenges of AI in Law | The emergence of artificial intelligence presents unprecedented challenges, introducing the risk of decisions based on false or biased data. | Must remain vigilant against manipulations and rigorously validate the submitted evidence. |
Liability Dilemma | Debates arise over whether liability rests with AI developers/providers or with the user who issues a fraudulent or incompetent prompt. | The judge must interpret the law in a balanced manner and distribute liability across all stages of information production and use. |
Need for Control Mechanisms | Corrective measures are crucial to penalize malicious actors (litigants or creators of defective prompts) as well as potential systemic failures. | The judicial authority must craft and apply guidelines and controls ensuring the integrity of legal proceedings. |
Symbiosis Judge–AI | AI can assist in identifying inconsistencies and analyzing large datasets, but it is not infallible. | The judge acts as a guarantor of truth, complementing AI’s analytical capacity with critical legal judgment and expertise. |
Broad Chain of Responsibility | Responsibility extends to developers, providers, users, and recipients, complicating accountability in case of error. | The judge must establish the origin of the illusion and determine appropriate compensation, ensuring fair distribution of liability. |
Proactive Technological Involvement | There is a possibility the judge might intervene ex officio in cases with massive impact on collective or diffuse rights, such as the dissemination of false information. | The judge becomes an active regulator, adapting the legal framework to technological challenges and protecting the public interest. |
Defense of Truth and Fundamental Rights | In an era where technological illusions can blur reality, the justice system must ensure AI is used ethically and within the law. | Beyond applying the law, the judge is the defender of truth and fundamental rights, ensuring technology is used for the common good. |
By leveraging these quantum principles, the code provides a more sophisticated tool for assessing the reliability and consistency of AI outputs—particularly valuable in critical applications where accuracy and truthfulness are paramount.
Note: The equations and code are conceptual and serve as a theoretical proposal.
14. EXECUTIVE SUMMARY;
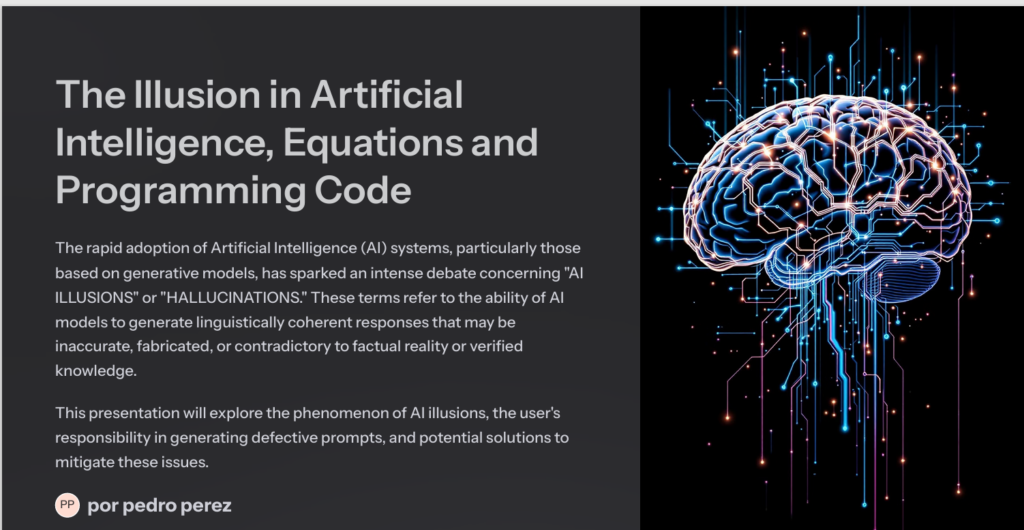
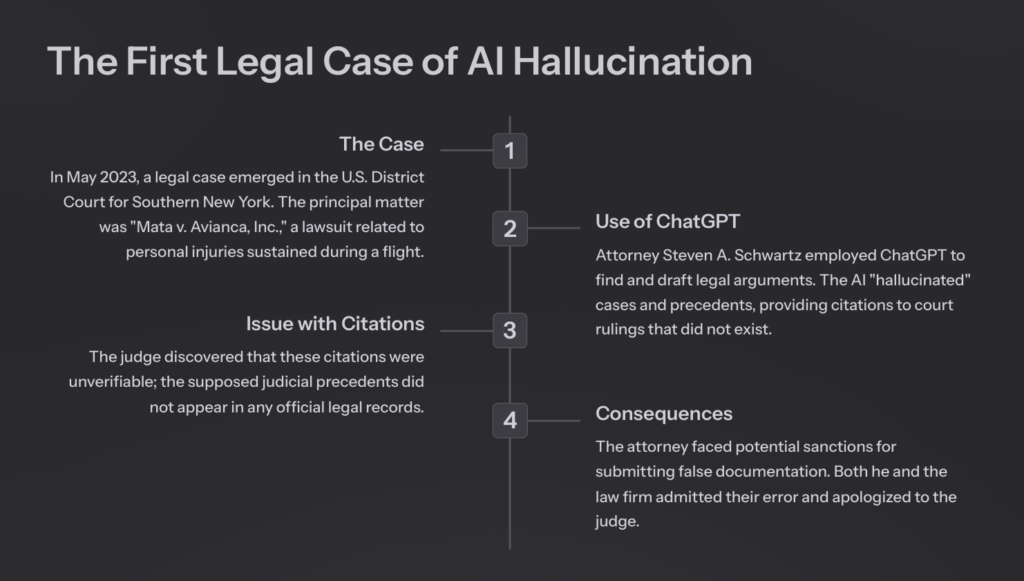
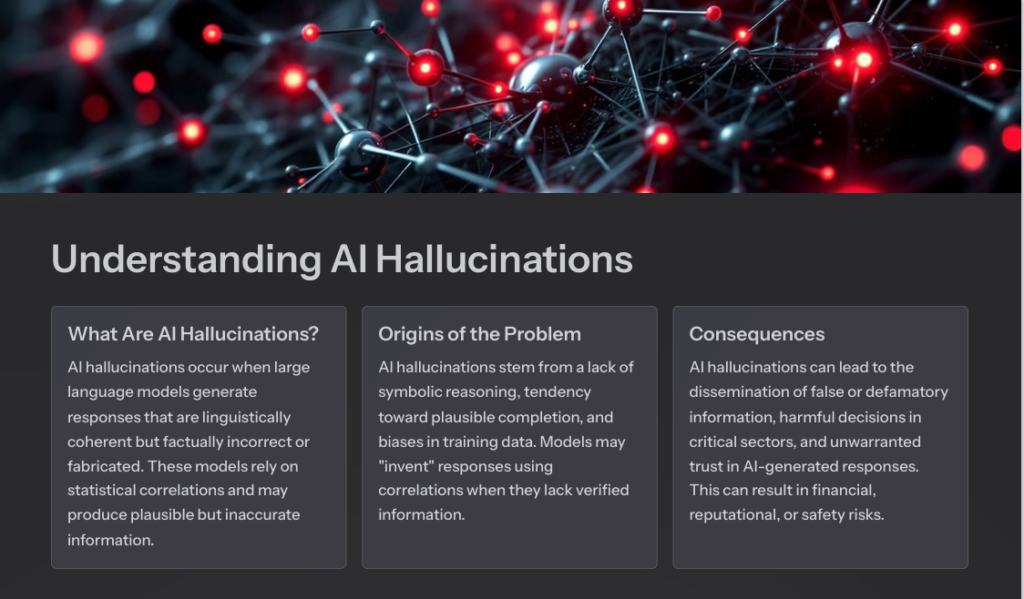
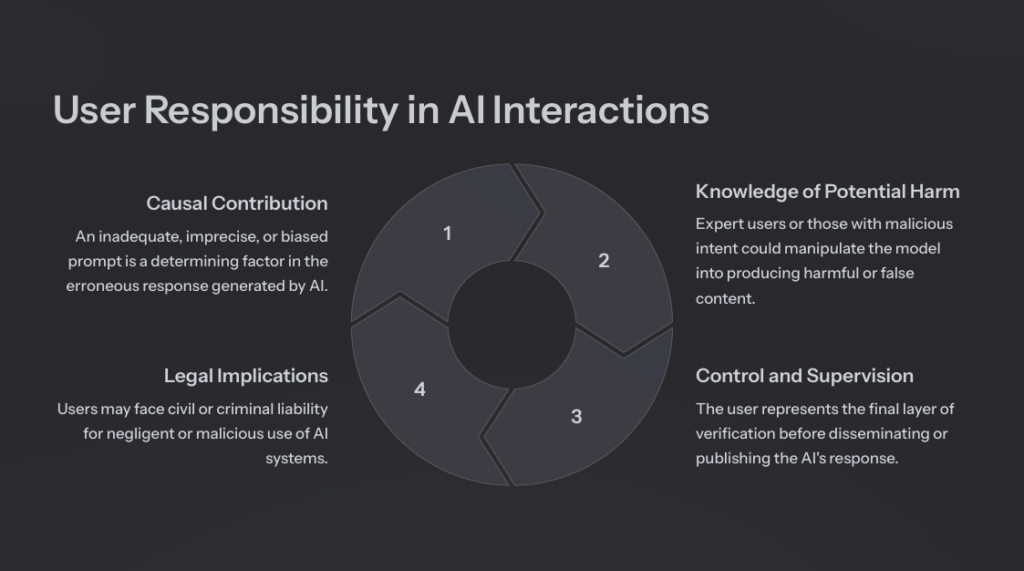
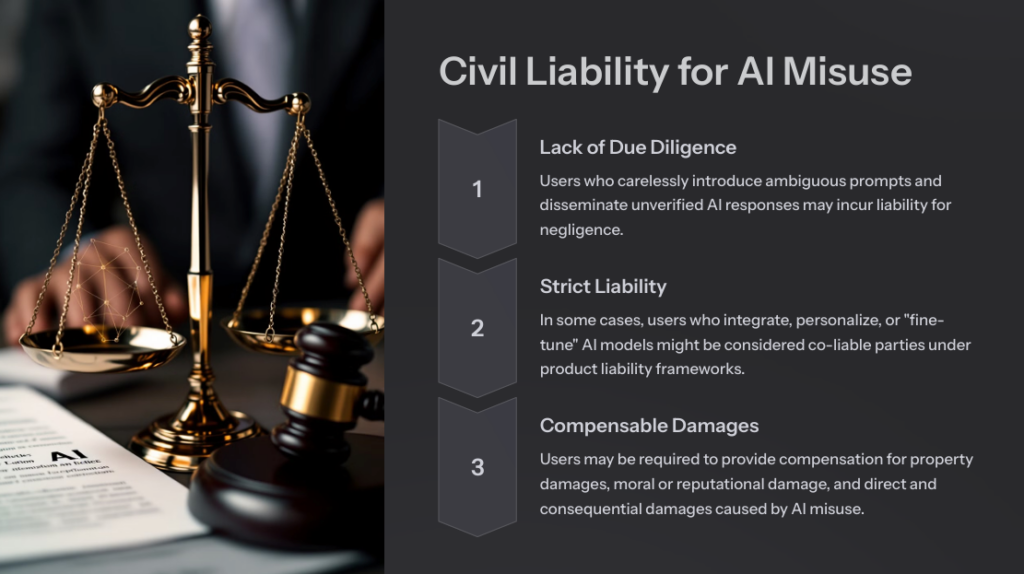
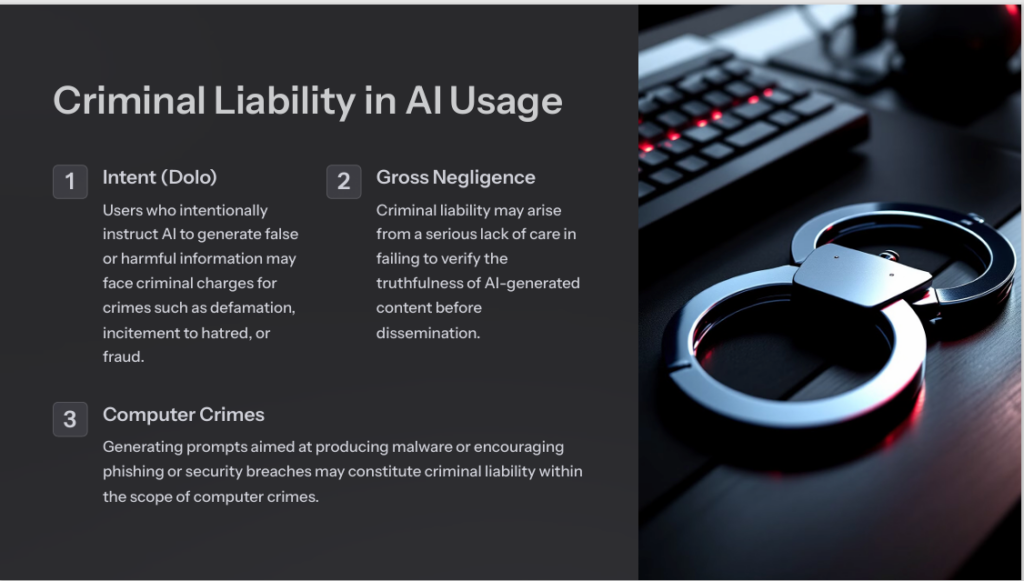
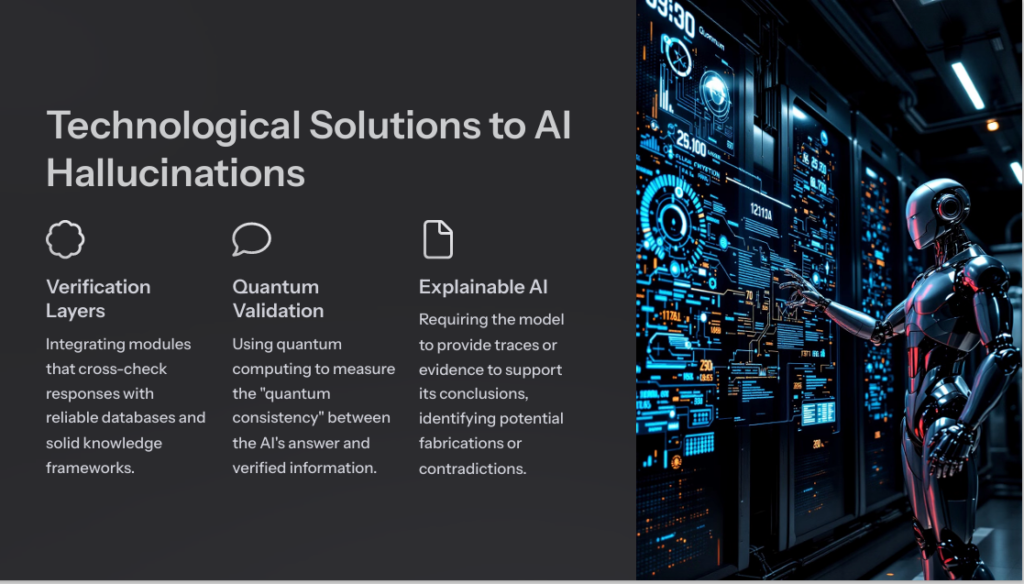
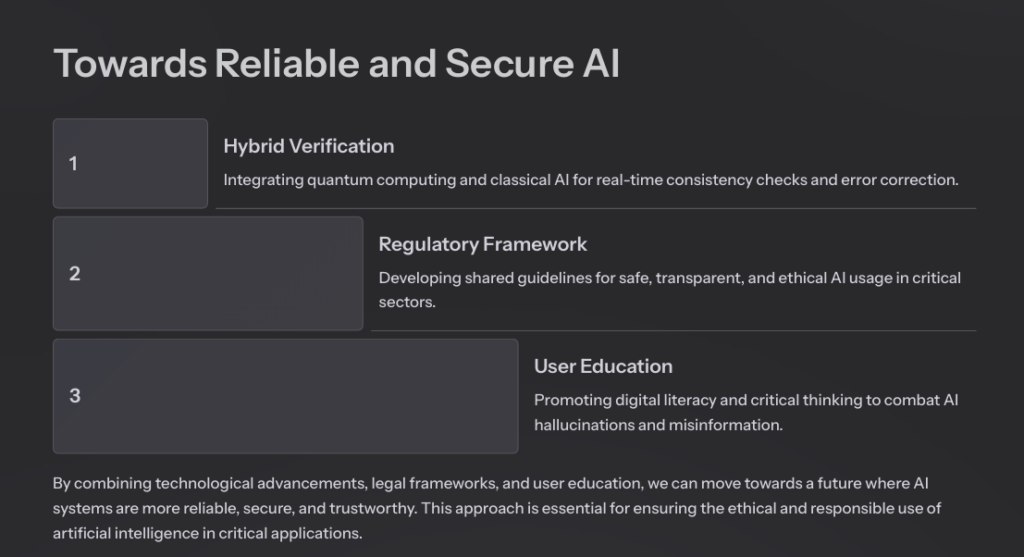
Final Observation:
This reference list covers both technical foundations (origin and treatment of AI hallucinations, fact-checking, quantum computing applications, etc.) and legal perspectives (civil and criminal liability for defective or negligent prompts, AI regulation at the international level). It also includes manuals of best practices and standards on transparency, verification, and ethics in AI usage.
Below is an integrated table in English that compiles all the key points from the context, including the introduction, main topics, additional tips and conclusions/suggestions ( Each row addresses a specific theme or section with columns for Description/Key Points, Risks/Challenges, Recommendations/Best Practices, and Examples/Applications when applicable.
TOPIC / SECTION | DESCRIPTION / KEY POINTS | RISKS / CHALLENGES | RECOMMENDATIONS / BEST PRACTICES | EXAMPLES / APPLICATIONS |
---|---|---|---|---|
Introduction: How to Become a Qualified Prompt Engineer | – It is essential to implement strategies and techniques for creating effective AI prompts and mitigating risks. Proper prompt engineering requires clarity in structure, recommendations, and examples to address key topics such as handling hallucinations. The goal is to become a “perfect prompt engineer” with solid AI skills. | – Lack of clear objectives can result in ineffective prompts and irrelevant outputs. Underestimating the importance of iterative testing and refinement. | – Align prompts with the specific objectives of your task (data retrieval, creative brainstorming, analysis, etc.). Continuously refine and validate outputs to mitigate hallucinations. Provide clear instructions regarding style, format, and desired content. | – Defining goals for a prompt-based assistant in customer service (e.g., reduce wait times, increase user satisfaction). Creating a structured approach to prompt engineering when launching a new generative AI feature. |
1. Elements of an Effective Prompt | – Clearly define the goal of your interaction with the AI. Avoid ambiguity; use concise language. – Specify structure, format, tone, and style. “Garbage in, Garbage out”: AI depends on prompt quality. | – Vague or ambiguous prompts can produce irrelevant or incorrect answers. – Lack of context can cause hallucinations or incomplete information. | – Be specific in your requests (e.g., “List 5 points…”). Provide examples if a particular style or format is desired. Clarify the expected output (text, list, table). – Review and refine prompts if the initial answer is unsatisfactory. | – Prompt: “Explain in 3 paragraphs why AI can hallucinate.” Prompt: “List 4 examples of successful corporate chatbots.” |
2. Prompt Drafting Process | – Step 1: Define your goal and expected outcome (information, analysis, creativity, etc.). Step 2: Draft and test the prompt. – Step 3: Analyze the response vs. the goal. – Step 4: Iterate until the desired quality is achieved. | – Skipping iteration may lead to settling for superficial or inaccurate answers. Underestimating the value of re-asking or deeper probing for refined responses. | – Embrace iteration: do not settle for the first result. Use follow-up questions or “chain-of-thought” prompts. – Ask the model to clarify its reasoning steps if needed. | – Prototyping prompts for risk analysis in business projects. Continuously refining advertising copy for a marketing campaign. |
3. Prompt Styles and Approaches | – Information Retrieval: Zero-shot, one-shot, few-shot, interview. Analysis/Reflection: Chain-of-thought, self-reflection, counterpoint. Creativity: Role-playing, simulated scenarios, tree-of-thought. Instructional: Step-by-step templates, guided narratives. Collaborative: Conversational, iterative prompts. | – Choosing the wrong style can delay accurate answers. Using a creative style when factual/technical data is needed may yield inconsistencies or hallucinations. | – Match the style to the objective and complexity of the topic. – Combine styles if appropriate (e.g., chain-of-thought + few-shot). | – Information Retrieval: “Show me 2022 sales data.” – Chain-of-thought: For complex step-by-step reasoning. Role-playing: For marketing ideas from a customer’s viewpoint. |
4. Handling AI Hallucinations | – AI may “invent” or fabricate information (hallucinations). – Causes include overgeneralizing from incomplete/biased data, or lack of context. – Also occurs if the model is poorly fine-tuned. | – Risk of wrong decisions based on false data. Legal/ reputational damage if misinformation is spread. | – Verify sources and compare answers with reliable documentation. – Retrain/recalibrate the model with high-quality data. Use chain-of-thought or self-reflection prompts to reveal reasoning. – Request references or detailed explanations for sensitive or critical information. | – Chatbots inventing return policies. – Text generators citing non-existent references. |
5. Evaluation & Validation of AI Responses | – Critical Thinking: Check factual accuracy, consistency, reliability, and bias. – Reflective Thinking: Ensure relevance to objectives, precision level, transparent reasoning, and ethics. | – Blind trust in AI can lead to costly mistakes.- Lack of transparency about how AI arrived at a conclusion. | – Ask for sources and verify them. Use common sense and professional experience. Evaluate alignment with business goals. Gather user feedback. | – Verifying strategic plan proposals with official reports or experts.Checking feasibility for new products (cost, practicality). |
6. Feasibility Factors & Use Case Design | – Use SMART goals (Specific, Measurable, Achievable, Relevant, Time-bound). Consider cost/benefit, operational feasibility, user impact, scalability, risks. – Align AI usage with business objectives (efficiency, revenue, customer experience). | – Underestimating integration costs, technical complexity, or staff training. – Overestimating user adoption without a clear engagement strategy. | – Define success metrics (ROI, time reduction, etc.). Check data quality and availability of experts. – Start with a prototype or pilot before scaling. | – Customer service chatbot aiming for a 30% cost reduction. Data-driven dynamic pricing for a 10% growth objective. |
7. Risks & Mitigation in AI Integration | – Common risks: poor data quality, hallucinations, security breaches, regulatory non-compliance, bias. – AI governance: responsibility, transparency, ethics. Cybersecurity and encryption to protect sensitive data. | – Reputational damage, financial losses, legal action. – Chain-reaction failures in third-party dependencies (vendors, APIs). | – Develop robust security and privacy policies. Regularly monitor and update models/software patches. – Involve ethics committees if impact is significant. Foster transparency and collaboration within the organization. | – Chatbots giving offensive or illegal information. Fines for breaching privacy regulations. |
8. Success & Failure Examples in Chatbots / GenAI | – Success: RideBikes, Inc. used a SMART goal (cost savings + user adoption). Invested in financial analysis, quality data, and iterative training. Failure: TechSupport Inc. quickly laid off staff without ensuring data quality or sufficient testing, resulting in legal costs and lost customers. | – Success: Clear ROI vision, iterative testing, balanced cost reduction vs. user satisfaction. – Failure: Unrealistic expectations, no validation, untrained staff. | – Success: Plan thoroughly, involve IT, management, users. – Failure: Avoided by scalable planning and human backup. – Seamless handoff from chatbot to human agents for complex issues. | – RideBikes cut 30% of staff gradually, achieved 40% chatbot usage. – TechSupport lost 10% of customers and faced lawsuits. |
9. AI Usage Metrics & Monitoring | – Define KPIs tied to business goals (e.g., shorter response times, higher sales, lower costs). Recalibrate the model periodically and measure accuracy. Track customer satisfaction. | – Without metrics, it’s impossible to assess AI performanceLack of continuous monitoring can let performance degrade unnoticed. | – Use dashboards and regular reporting systems. – Define clear metrics: adoption rate, error rate, ROI, customer satisfaction. – Update the model regularly to maintain effectiveness. | – Chatbot measuring % of successful interactions without human intervention. – Text generator tracking CTR in marketing campaigns. |
10. Scalability & Continuous Adaptation | – AI should scale with the organization’s growth. – Consider infrastructure costs (cloud, servers, maintenance). Plan for integration with legacy or future systems. | – Underestimating user demand can overload servers, harming service quality. Lack of updates makes the model obsolete. | – Plan incremental growth (start with MVP/pilot). Negotiate cloud capacity for peak usage. Regularly update the model and conduct performance audits. | – E-commerce sites experiencing spikes on Black Friday. Recommendation systems with expanding user bases. |
11. AI Governance & Ethical Aspects | – Governance ensures responsibility and transparency. Anticipate ethical risks (discrimination, biases, automated decisions affecting vulnerable groups). – Importance of cross-department collaboration and clear user communication. | – Lack of accountability can lead to abuses and reputational damage. – Emerging regulations (GDPR, responsible AI standards) requiring compliance. | – Create AI policies and ethics committees for reviews. Disclose when users interact with AI. – Assign clear accountability (AI governance roles). – Stay updated on new laws and regulations. | – Companies with “Chief AI Officers” or ethics committees. Transparent chatbots: “I am a virtual assistant, not a human.” |
12. Conclusions & Final Recommendations | – Align technical capabilities (prompting, data, training) with business goals for successful generative AI. – Quality prompts and answer validation are crucial to avoid hallucinations. – Invest time in solid use cases (objectives, metrics, scaling plan). | – Implementing AI without a plan or incremental testing can lead to expensive failures. Underestimating human oversight and updates causes outdated or misleading results. | – Combine critical thinking with proper prompt engineering. Iterate, validate, and retrain AI as goals and data evolve. Maintain a holistic view: security, scalability, finances, user experience. – Include feedback loops for continuous improvement. | – Piloting AI projects before organization-wide deployment. Integrating chatbots with CRM/analytics to measure real business impact. |
8.6 Key Points & Tips to Become a “Perfect Prompt Engineer” | 1. Know Your Objective: Understand precisely what you need (data, ideas, analysis, style). 2. Clear Structure: Indicate desired response format (list, table, paragraphs) and tone (formal, creative, concise). 3. Include Examples: Use one-shot/few-shot prompting to illustrate the target output. 4. Iterate & Refine: Adjust prompts if results are off-target. 5. Constant Verification: Validate with reliable sources, check consistency, ask for explanations if hallucinations appear. 6. Update & Improve: Retrain the model with current data; evolve prompts as goals shift. | – Risk: Without a clear objective and structured prompts, AI outputs can be low-quality or irrelevant. Risk: Failing to refine or verify leads to persistent inaccuracies and possible misinformation. | – Provide context and examples to reduce ambiguity. Continuously refine instructions to improve accuracy and relevance. Employ follow-up questions to clarify or deepen the content. Keep prompts up-to-date with changing objectives or new data. | – Prompt Example: “Based on the latest market trends, list 3 innovative product ideas for the coming year.” – Iterative Approach: Starting with a broad creative prompt and narrowing down the details in subsequent prompts. |
8.7 Conclusions & Suggestions | – Reduce Hallucinations: Provide enough context/information before asking for resolutions. – Inverted Interaction Pattern: Have the AI ask the user about their role, preferences, or key data first. – Personalization: Use FAQs, set response styles, or offer action menus to avoid generic or incorrect replies. – FAQ Methodology: Organize common questions/answers to save time, provide clarity, and simplify user support. Validate & Cite: Always validate information, cite references, and redirect to human help if ambiguities arise. | – Risk: Omitting essential context can increase hallucinations. – Risk: Overlooking user preferences or failing to personalize leads to generic or irrelevant replies. | – Collect frequent questions in a single FAQ to streamline user queries. – Prompt the AI to confirm user details or context before generating answers. – Provide references or further reading links when necessary. – Have escalation paths for complex queries that AI alone can’t handle. | – Chatbot Onboarding: Asking “What kind of assistance are you looking for today?” before offering responses. FAQ-Driven Support: A structured menu for frequently asked technical questions in a product support portal. |
By following these structured insights, you can enhance your skills as a “perfect prompt engineer,” effectively mitigate hallucinations, and develop robust AI use cases aligned with strategic business goals.
15. BIBLIOGRAPHY:
Reference / Citation | URL or Link | Brief Note |
---|---|---|
Bender, E., & Koller, A. (2020). “Climbing towards NLU: On Meaning, Form, and Understanding in the Age of Data”. ACL. | Topic: limitations of large language models and their hallucinations; presented at the Association for Computational Linguistics (ACL) conference. | |
Maynez, J., Narayan, S., Bohnet, B., & McDonald, R. (2020). “On Faithfulness and Factuality in Abstractive Summarization”. ACL. | https://aclanthology.org/2020.acl-main.173 | Topic: generation of fabricated (hallucinated) information during text summarization; conference main session. |
Ji, Z., Lee, N., Fries, J., et al. (2023). “Survey of Hallucination in Natural Language Generation”. ACM Computing Surveys, 55(12). | https://doi.org/10.1145/3571730 | Topic: general overview and taxonomy of “hallucinations” in natural language generation. |
Thorne, J., Vlachos, A., Christodoulopoulos, C., & Mittal, A. (2018). “FEVER: a Large-scale Dataset for Fact Extraction and VERification”. NAACL. | https://aclanthology.org/N18-1074 | Resource for automatic fact-checking, helpful in detecting fabricated AI information. |
Guo, Q., Tang, X., Duan, N., et al. (2021). “LongT5: Efficient text-to-text transformer for fact checking”. EMNLP. | https://arxiv.org/abs/2112.07916 | Preprint in arXiv, optimizing approaches for factual verification and reducing inaccurate outputs. |
European Commission. (2021). “Proposal for a Regulation … (AI Act)”. | https://eur-lex.europa.eu/legal-content/EN/TXT/?uri=CELEX:52021PC0206 | Topic: EU legislative framework on AI and responsibilities of users/providers. |
OECD. (2019). “OECD Principles on Artificial Intelligence”. | https://legalinstruments.oecd.org/en/instruments/OECD-LEGAL-0449 | International guidelines for responsible use of AI. |
Montagano, M. (Ed.). (2020). AI Liability: The New Ecosystem of Risk. Cambridge University Press. | https://www.cambridge.org/core/books/ai-liability-the-new-ecosystem-of-risk/ | Topic: emerging civil and criminal liability in AI environments. Subscription required. |
Mata v. Avianca, Inc., No. 22-cv-1461 (S.D.N.Y. May 2023). | https://casetext.com/case/mata-v-avianca-inc | Topic: real case where a lawyer submitted nonexistent legal citations generated by ChatGPT. Illustrates the issue of “hallucinated” citations and professional liability for not verifying them. |
Restatement (Third) of Torts: Product Liability (American Law Institute). | https://www.ali.org/publications/show/torts-product-liability/ | Foundational document in the U.S. for assessing civil liability of manufacturers (including potential “producers” of software). |
Spivak, D. (2022). “Negligence and AI Tools: A New Frontier in American Tort Law”. Harvard Journal of Law & Technology, 35(3). | https://jolt.law.harvard.edu/ | Topic: user negligence in configuring/using AI tools and resulting legal consequences. |
Supreme Civil Chamber (Venezuela), 24-02-2015, Exp. Nº 14-367. | http://www.tsj.gob.ve/ | Reiterates civil liability for wrongful acts under Art. 1,185 of the Civil Code; the official platform for Venezuelan court rulings. |
Law on Data Messages and Electronic Signatures (Venezuela, Official Gazette No. 37,148, 2001). | http://www.tsj.gob.ve/legislacion/ley-de-mensajes-de-datos-y-firmas-electronicas | Topic: legal validity of electronic documents and duty of care; also relevant to AI-generated content. |
Organic Law on Science, Technology and Innovation (LOCTI), Official Gazette No. 39,575, 2010 (Venezuela). | http://www.tsj.gob.ve/ search recommended) | While not directly regulating AI, it establishes legal principles and liability for technological initiatives. |
Special Law against Computer Crimes (Venezuela). | https://cndc.mijp.gob.ve/marco-legal/ley-especial-contra-los-delitos-informaticos/ | Topic: penal framework for tech-related crimes (malicious content distribution, etc.). |
Kerr, I. (Ed.). (2019). Cybercrime and Digital Evidence: Cases and Materials. West Academic Publishing. | https://www.westacademic.com/ | Analysis of cybercrime and digital evidence, applicable to AI and algorithmic liability. |
Smit, S. (2021). “Criminal Liability for AI-Generated Content: The User’s Mens Rea”. Journal of Robotics, Artificial Intelligence & Law, 4(1). | https://www.wolterskluwer.com/ | Topic: criminal responsibility for illicit content generated by AI, focusing on user’s intent (dolo) or gross negligence. |
Gunning, D. (2017). “Explainable Artificial Intelligence (XAI)”. DARPA. | https://www.darpa.mil/program/explainable-artificial-intelligence | Development of explainable AI to detect potentially biased or false outputs. |
Doshi-Velez, F., & Kim, B. (2017). “Towards A Rigorous Science of Interpretable Machine Learning”. arXiv:1702.08608. | https://arxiv.org/abs/1702.08608 | Preprint formalizing methodologies for making ML models more transparent and traceable. |
Wittek, P. (2019). Quantum Machine Learning: What Quantum Computing Means to Data Mining. Academic Press. | https://www.elsevier.com/books/quantum-machine-learning/wittek/978-0-12-800953-6 | Topic: application of quantum computing to improve ML algorithms. |
Rebentrost, P., Lloyd, S., & Slotine, J. J. (2019). “Quantum Machine Learning for Classical Data”. Nature Physics, 15(11). | https://doi.org/10.1038/s41567-019-0648-8 | Demonstrates how quantum computing can compare information states and detect inconsistencies. |
Chiang, C. F., Laudares, F., & Esteves, T. (2021). “Applying Swap Test for Consistency Checks in Quantum-Enhanced NLP Systems”. Quantum Information & Computation, 21(5). | https://www.rintonpress.com/journals/qic-archive.html | Explains the “swap test” to measure fidelity between AI responses and factual data. |
Anderson, B., & McGrew, R. (2019). “Cybersecurity Data Science: An Overview from Machine Learning Perspective”. Journal of Information Security and Applications, 46. | https://doi.org/10.1016/j.jisa.2019.05.002 | Topic: applying ML techniques to cybersecurity, relevant for countering malicious AI. |
Brundage, M., Avin, S., Wang, J., & Krueger, G. (2018). “The Malicious Use of Artificial Intelligence: Forecasting, Prevention, and Mitigation”. Future of Humanity Institute, Oxford. | https://arxiv.org/abs/1802.07228 | Pioneering paper on emerging AI risks, proposing countermeasures (counter-AI). |
ISO/IEC 27032:2012. “Information technology – Security techniques – Guidelines for cybersecurity”. | https://www.iso.org/standard/44375.html | International standard guidelines for protecting systems from cyber threats, applicable to AI counterintelligence. |
High-Level Expert Group on Artificial Intelligence (2019). “Ethics Guidelines for Trustworthy AI”. European Commission. | https://digital-strategy.ec.europa.eu/en/library/ethics-guidelines-trustworthy-ai | Topic: principles of transparency, robustness, and responsibility in AI. |
Jobin, A., Ienca, M., & Vayena, E. (2019). “The Global Landscape of AI Ethics Guidelines”. Nature Machine Intelligence, 1(9). | https://doi.org/10.1038/s42256-019-0088-2 | Global comparison of ethical guidelines in AI. |
Floridi, L., & Taddeo, M. (2018). “What is Data Ethics?”. Philosophical Transactions of the Royal Society A, 374(2083). | https://doi.org/10.1098/rsta.2016.0360 | Topic: responsibility in managing information in AI systems, relevant for preventing the spread of false data. |
IBM. (2022). “AI Governance Framework: A Guide for Building Responsible, Transparent, and Accountable AI Systems”. | https://www.ibm.com/blogs/policy/ai-governance-framework/ | Recommendations for multi-stage reviews and best governance practices in AI. |
ISO/IEC TR 24028:2020. “Information Technology—Artificial Intelligence—Overview of Trustworthiness in AI”. | https://www.iso.org/standard/77608.html | Technical guidance on evaluating the reliability and credibility of AI. |
Microsoft. (2021). “Responsible AI Standard”. | https://www.microsoft.com/ai/responsibleai | Internal guidelines on AI design and usage, validation, and user accountability. |
Daubert v. Merrell Dow Pharmaceuticals, Inc., 509 U.S. 579 (1993). | https://supreme.justia.com/cases/federal/us/509/579/ | U.S. Supreme Court decision setting the reliability standard for scientific evidence, applicable to AI-based tools. |
Schellekens, M., & Kurtz, P. (2022). “Product Liability 2.0: Revisiting the Negligence Standard in the AI Era”. Stanford Technology Law Review, 25(2). | https://law.stanford.edu/stanford-technology-law-review/ | Argues for expanding the negligence standard to users who modify or train AI systems. |
European Union Agency for Cybersecurity (ENISA). (2021). “Securing Machine Learning Algorithms”. | https://www.enisa.europa.eu/publications/securing-machine-learning-algorithms | Guidelines for detecting and mitigating manipulation or hallucinations in ML systems. |
Prepared by: PEDRO LUIS PEREZ BURELLI
© Copyright (Authorship)
PEDRO LUIS PÉREZ BURELLI / perezburelli|@gmail.com / perezburelli@perezcalzadilla.
https://www.linkedin.com/in/pedro-luis-perez-burelli-79373a97/
Thanks I have just been looking for information about this subject for a long time and yours is the best Ive discovered till now However what in regards to the bottom line Are you certain in regards to the supply.
«This article is real